Progress towards accurate prediction of overall survival in men with metastatic castration-resistant prostate cancer
Prostate cancer (PC) is the most frequently diagnosed male malignancy and the 2nd or 3rd leading cause of cancer deaths in men in the developed countries. The disease progresses from locally invasive carcinoma to metastatic prostate cancer (mPC). While PC metastasizes to the liver and lung, bone is the most frequent site of PC metastasis. Distant metastasis likely marks the point of no return progress towards the worst prognosis. Owning to the landmark discovery that metastatic PC requires androgen receptor (AR) signaling by Charles Huggins in 1941, androgen deprivation therapy (ADT) remains the standard of care for mPC patients. Although the treatment provides initial benefits in the majority of patients with mPC, metastatic castration-resistant prostate cancer (mCRPC) inevitably arises. Prior to 2011, docetaxel was the only second line-therapy (1), and prolonged median overall survival (OS) in patients with mCRPC by 3 months. Since then, the second generation anti-androgens (abiraterone and enzalutamide), radium-223, cabazitaxel, and Sipuleucel-T have become available in the clinic. Although these therapies are not curative, they extend OS in patients with mCRPC (2). As these drugs have different mechanisms of action, they could be used in a variety of combinations either sequentially or simultaneously to maximize benefits to patients with mPC or mCRPC. For example, ADT plus docetaxel is superior to either alone (3) and is becoming the new standard of care for patients with mPC with good performance status. Clearly, improving our knowledge on the course of mCRPC will contribute to the development of rational treatment plans with the currently available medicines and thereby improves patient management. In this regard, identifying parameters to accurately predict survival of patients with mCRPC is an area of active research; there are 113 and 40 published studies related to the topic of mCRPC and prognostic biomarkers or prognostic models in PubMed (https://www.ncbi.nlm.nih.gov/pubmed/advanced) up to Dec 3, 2016. In order to yield a robust predictive model, it will be essential for a team with combined expertise in clinic and machine learning to analyze comprehensive sets of clinical data.
This effort was recently reported (4). Using the Dialogue for Reverse Engineering Assessments and Methods (DREAM) challenge platform through Project Data Sphere, Guinney and colleagues analyzed the impact of more than 150 clinical baseline variables on OS of 2,336 mCRPC patients with an array of state-of-the-art machine learning tools including an ensemble of penalized Cox regression (ePCR) model, and formulated a powerful model for predicting OS of mCRPC patients (4). More importantly, the study was a part of an even much larger and comprehensive effort: sharing and analyzing the clinical data by 163 experts consisting of 50 independent teams worldwide (4). The clinical data used were compiled from the control arms of five large randomized phase III clinical trials through the effort of Project Data Sphere (n=2,336, Table 1). Except ENTHUSE M1, patients in the comparator arms from the rest of clinical trials were treated with docetaxel (Table 1). More than 150 clinical variables provided in individual clinical trials were centrally curated by the organizers of the DREAM challenge to yield a core table; of which data from three clinical trials (n=1,600) were distributed to 50 teams for training analysis, and data from ENTHUSE 33 and ENTHUSE M1 were used to score a winning ePCR model and validate the model (Figure 1) (4).
Table 1
Cohortb | Number | Treatment received | Application | Livere | Kidneye |
---|---|---|---|---|---|
ASCENT2 | 476 | Doc + prednc | Training | Normal | Normal |
MAINSAIL | 526 | Doc + predn + placebo | Training | Adequatef | Adequateg |
VENICE | 598 | Doc + predn + placebo | Training | Adequate | Adequate |
ENTHUSE 33 | 470 | Doc + placebo | Test and scored | Adequateh | Adequatei |
ENTHUSE M1 | 266 | Placebo | Validation | Adequate | Adequate |
a, mCRPC patients; b, all cohorts were from the control arms of the indicated phase III clinical trials; c, Doc/docetaxel and predn/prednisone (prednisone or prednisolone for VENICE); d, a subgroup (n=157) was used for testing individual models and a second subgroup (n=313) was for predicting OS (see Figure 1 for details); e, liver and kidney function at the time of patient recruitment; f, total bilirubin <1xULN (up limit of normal), AST and/or ALT (alanine aminotransferase) <1.5×ULN; g, creatinine clearance >50 mL/minute; h, patients with ALT or AST >2.5×ULN in the presence of liver metastasis were excluded; i, patients with 24 hours creatinine clearance <50 mL/min were excluded. DREAM, Dialogue for Reverse Engineering Assessments and Methods.
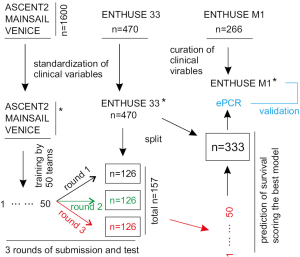
The model performances well for stratifying low and high risk patients in both ENTHUSE 33 (n=313) and ENTHUSE M1 (n=226) cohorts (Figure 1) with predictive accuracy determined by iAUC (integrated time-dependent area under the curve) of respectively 0.791 and 0.768 (4). Both risk groups have a significant difference in OS as analyzed by the Kaplan-Meier method: hazard ratio (HR) 3.32, 95% confidence intervals (CI): 2.39–4.62, P<0.0001 for ENTHUSE 33 and HR 2.86, 95% CI: 2–4.12, P<0.0001 for the control arm of ENTHUSE M1 (4). Unlike patients in the control arms of other four clinical trials, patients from the comparator arm of ENTHUSE M1 were receiving only placebo (Table 1) (4). Validation of the ePCR model in the latter cohort confirmed its predictive value as disease- rather than treatment-dependent.
The ePCR model not only won the challenge amount 50 competitive teams but also outperformed a prognostic model recently published by Halabi and colleagues (5) in stratifying low and high risk patients in both ENTHUSE 33 and ENTHUSE M1 (4). The Halabi model holds superiority to similar models published prior to their research (5); ePCR is thus likely the best prognostic platform currently available in the public domain to predict OS of mCRPC patients. However, this comes with no surprise, as the DREAM challenge also outmatched the Halabi group in terms of patient resources, team size (50 teams and 163 individuals) and collective expertise, as well as state-of-the-art machine learning and statistical modelling methods (4). While the Halabi model incorporates 22 clinical variables using the adaptive least absolute shrinkage and selection operator (LASSO) penalized Cox regression, ePCR analyzed 150 clinical variables using an advanced Cox regression model: an ePCR (4,5). Nonetheless, the Halabi model was able to stratify very similar groups of low or high risk patients from ENTHUSE 33 compared to the ePCR model (4), and is a much simpler model than ePCR. Importantly, the Halabi model is available on line as a reference tool for physicians to evaluate their mCRPC patients (https://www.cancer.duke.edu/Nomogram/firstlinechemotherapy.html.); the model thus has its applications. Likewise, Guinney and colleagues will likely make their model available in the same manner in the near future. Nonetheless, it is clear that the ePCR model is more comprehensive compared to the Halabi model. The study thus has set a new standard of data sharing and analyzing in model-building. This is particularly beneficial for clinical trials not only because of the massive effort that has been spent on these trials but also due to immediate benefits that it can bring to patients.
In addition to producing a robust prognostic model, the data sharing effort also provides novel knowledge in the prediction of OS for mCRPC patients. Aspartate aminotransferase (AST) was indicated as an important prognostic biomarker (4). However, most patients were recruited to these clinical trials with normal or adequate liver function (Table 1). The prognostic potential of AST might be resulted from liver injury caused by liver metastasis, as 1–14% of patients in all five clinical trials used in this study had liver metastasis (4). Hepatic metastasis is a well-known cause for poor outcome. It will be interesting to examine AST’s prognostic values in patients with only bone metastasis or lack of liver metastasis. The same concern also applies to kidney function. Patients were recruited also with adequate renal function (Table 1); whether the renal functional measurements (creatinine, creatinine clearance, and calculated creatinine clearance) display prognostic values should be further investigated. Will it be possible that the prognostic values of these kidney criteria reflect their interactions with other hematologic markers? As Guinney and colleagues acknowledged that these interactions did not reach a significant level (4), the network contributions to the evaluation of OS in patients with mCRPC, as implied in this report, should be explored in future.
Nonetheless, the concept of network of interaction in predicting OS for mCRPC patients is intriguing. The network involves biomarkers derived from the immune, liver, and renal systems (4). While the prognostic contributions of immunologic biomarkers are not surprising, how renal functions impairment contributes to mCRPC progression remains unclear. Will insufficient filtration of some compounds contribute to mCRPC progression? More attention has been paid to search for tumor-derived prognostic factors. Guinney and colleagues raise an interesting issue about the impact of the patient overall health condition on the deadly progression of mCRPC. Furthermore, work on the impact of the heterogeneities in individual tumors versus individual host conditions in mCRPC progression may reveal novel insights.
Answering the above questions will certainly requires extensive and open data sharing and collaborative research efforts. Furthermore, this type of big-data analyses needs to incorporate molecular events. For example, prostate cancer stem cells (PCSCs) are the driving force in PC evolution; potential PCSC biomarkers should be considered. Will the tumor samples in the clinical trials used in the study by Guinney et al. be available for profiling gene expression changes using RNA sequencing and genomic alterations through whole genome sequencing? If not, future studies should be coordinated at the levels of RNA and DNA alterations.
Acknowledgments
Funding: D Tang is supported by grants from Teresa Cascioli Charitable Foundation Research Award in Women’s Health, Canadian Breast Cancer Foundation, and Cancer Research Society.
Footnote
Provenance and Peer Review: This article was commissioned and reviewed by the Section Editor Longfei Liu, MD, PhD (Department of Urology, Xiangya Hospital, Central South University, Changsha, China) and Assistant Editor Yongbao Wei, MD (Department of Urology, Fujian Provincial Hospital, the teaching hospital of Fujian Medical University, Fuzhou, China).
Conflicts of Interest: All authors have completed the ICMJE uniform disclosure form (available at http://dx.doi.org/10.21037/jxym.2017.02.10). The authors have no conflicts of interest to declare.
Ethical Statement: The authors are accountable for all aspects of the work in ensuring that questions related to the accuracy or integrity of any part of the work are appropriately investigated and resolved.
Open Access Statement: This is an Open Access article distributed in accordance with the Creative Commons Attribution-NonCommercial-NoDerivs 4.0 International License (CC BY-NC-ND 4.0), which permits the non-commercial replication and distribution of the article with the strict proviso that no changes or edits are made and the original work is properly cited (including links to both the formal publication through the relevant DOI and the license). See: https://creativecommons.org/licenses/by-nc-nd/4.0/.
References
- Mukherji D, Omlin A, Pezaro C, et al. Metastatic castration-resistant prostate cancer (CRPC): preclinical and clinical evidence for the sequential use of novel therapeutics. Cancer Metastasis Rev 2014;33:555-66. [PubMed]
- Agarwal N, Di Lorenzo G, Sonpavde G, et al. New agents for prostate cancer. Ann Oncol 2014;25:1700-9. [Crossref] [PubMed]
- Sweeney CJ, Chen YH, Carducci M, et al. Chemohormonal Therapy in Metastatic Hormone-Sensitive Prostate Cancer. N Engl J Med 2015;373:737-46. [Crossref] [PubMed]
- Guinney J, Wang T, Laajala TD, et al. Prediction of overall survival for patients with metastatic castration-resistant prostate cancer: development of a prognostic model through a crowdsourced challenge with open clinical trial data. Lancet Oncol 2017;18:132-142. [Crossref] [PubMed]
- Halabi S, Lin CY, Kelly WK, et al. Updated prognostic model for predicting overall survival in first-line chemotherapy for patients with metastatic castration-resistant prostate cancer. J Clin Oncol 2014;32:671-7. [Crossref] [PubMed]
Cite this article as: Mei W, Kapoor A, Major P, Shayegan B, Tang D. Progress towards accurate prediction of overall survival in men with metastatic castration-resistant prostate cancer. J Xiangya Med 2017;2:17.