Identification of forward regulated hub genes and pathways for cancer stem cell characteristics in African American breast cancer by the network analysis
Introduction
Breast cancer is the leading cause for the death of a woman and characterized by a high incidence, poor curativeness and heterogeneity. Its incidence and survival status are different among different ethnic groups (1). Compared with white people, African American are more likely to develop breast cancer and African American women with breast cancer present a higher proportion of macrophage cell infiltration and a worse prognosis (2,3). Triple-negative breast cancer is more common in younger African American women and they tends to die within 2 years after the diagnosis (4). Currently, surgery combined with postoperative chemotherapy, radiotherapy or endocrine therapy is the first choice for breast cancer, but postoperative treatment methods for triple-negative breast cancer are still quite limited. Postoperative recurrence and metastasis are the main causes for the failure of breast cancer treatment.
With the continuous development of oncology, cancer stem cells (CSCs) have been found in different solid malignant tumors (5-7). Although CSC only accounts for a small part of tumor cells, it plays an important role in the process of tumorigenesis, development, invasion and metastasis (8). CSC studies focus on the mechanisms of the development, progression, metastasis, recurrence and drug resistance of breast cancer (9). mRNAsi is used to explore the similarity index between tumor cells and stem cells. The index which is closer to one indicates the lower degree of cell differentiation and the stronger characteristics of stem cells. With the screened key genes closely related to the characteristics of tumor stem cells through mRNAsi, tumor stem cell clusters can be easily screened and the molecular mechanisms regulating the growth, differentiation and cell migration of tumor stem cells can be further understood, thus improving the traditional tumor treatment strategies.
In this study, mRNAsi was used to screen key genes and signaling pathways related to tumor stem cells, and Oncomine was used to verify the differential expression of screened hub genes in different tissues and their correlation with her-2, estrogen receptor and progesterone receptor. In addition, the influences of hub genes on chemotherapy sensitivity was analyzed. The study provides the basis for understanding the biological behaviors of African American breast cancer stem cells (BCSCs) and exploring new targeted therapies.
Methods
The study was conducted in accordance with the Declaration of Helsinki (as revised in 2013). All analyses were based on previous published data, thus no ethical approval and patient consent are required. The source of the data is clearly indicated in the article.
Downloading and processing data
In TCGA database (https://portal.gdc.cancer.gov/), after setting the search criteria, African American breast cancer RNA-sequencing (RNA-seq) data were downloaded. The clinical data of six normal samples, 174 breast cancer samples and 177 breast cancer patients were obtained, and the data without follow-up records were excluded. The raw data were transformed into Ensembl ID and the scripts were merged with Perl Language.
From the GEO database (https://www.ncbi.nlm.nih.gov/geo/) to download GSE142102 and GSE86374 data, obtain African American and Mexican women gene expression in patients with breast cancer. Among them, there were 226 cases of African American women with breast cancer, and 159 cases of Mexican breast cancer, including 35 cases of adjacent tissues and 124 cases of cancer tissues. Difference analysis was conducted on two microchips respectively to obtain the different genes of African American women with breast cancer. After eliminating the batch effect, the two sets of GSE data showed no statistical difference.
Differential expression of mRNAsi and its correlation with survival time
We used GraphPad to explore the prognostic value of mRNAsi. First, the difference in mRNAsi expression between normal tissues and cancer lesions was analyzed by log-rank tests, and then the cancer cases were divided into two groups (the low expression group and the high expression group) with the median mRNAsi score as the reference value. Survival analysis was performed with the data of the two groups to analyze the correlation of mRNAsi with survival time.
Differential gene screening and co-expression analysis
The differential expressions of the samples in the normal group and the cancer group were analyzed in the “edgeR” R package. Differential gene screening criteria were set as: |logFC| ≥1 and false discovery rate (FDR) <0.05. Exclusion criteria were set as: gene expression less than one. The expression level of the same gene was averaged. The differential genes expressed jointly by TCGA and GEO were obtained by making Venn diagram. The selected differential genes were displayed with heat map and volcano map. The co-expression of mRNAsi-related genes was analyzed by WGCNA R package as follows. Firstly, the differential gene expression data were read. The normal sample expression values were removed and the genes without ID were deleted. The samples were clustered and dispersed samples were deleted. Then the mRNAsi expression data were read and the intersection with differential genes was obtained. The samples were clustered again. The range of the pseudo-power index was 1:20. The distances between genes were calculated and then converted into topological overlap matrix (TOM) for the gene clustering analysis and identification of dynamic shear modules. Each module contained at least 50 genes. Less than 0.25 was used as the filter threshold of differential genes for clustering similar module and merging similarity modules. The differences between modules were analyzed to further explore the correlations between genes and modules, and the correlations between genes and mRNAsi (GS, gene significance, Measure the correlation between genes and sample traits) to display the scatter plots of mRNAsi and modules for screening key genes.
Analysis of key genes
After the co-expression analysis of WGCNA, key genes were extracted from the modules of interest, and the threshold of screening key genes was set as: P value <0.01, cor. Gene MM >0.6 and cor. Gene GS >0.5. The differential expression of key genes in normal tissues and breast cancer tissues were used compared in the ggpubr R package and the pheatmap R package, and the Pearson’s correlation between key genes were analyzed in the Corrplot R package. The PPI network of key genes was constructed with the STRING database and the combined score >0.4 in the interactions between proteins was considered to be statistically significant. After downloading corresponding string_interactions.tsv file, the number of adjacent nodes of each gene was calculated and the hub genes were analyzed.
Characterizing hub genes by GO enrichment, KEGG pathway analysis
GO enrichment and KEGG pathway analysis were performed for functional and pathway enrichment. The bar chart and bubble chart showed the results of GO enrichment and KEGG enrichment analysis of the first 30 groups with statistical differences. Filter thresholds were set as follows: modified P value <0.01 and q value <0.05.
Data validation
The microarray database Oncomine (http://www.oncomine.org) was used for the analysis of the differential expression of hub genes between normal tissues and cancer tissues and the correlations between hub genes and other indices (her-2, ER, PR status and response to chemotherapy) were discussed. The filter conditions was set as: cancer type: breast cancer, analysis type: cancer vs. normal analysis, treatment: chemotherapy treatment.
Results
Differential expression of mRNAsi and survival analysis
mRNAsi can indicate the similarity between tumor cells and stem cells and evaluate the proliferation and differentiation abilities of tumor stem cells. In this study, 1,188 mRNAsi expression samples were extracted, including 98 samples from normal tissues and 1,090 samples from breast cancer tissues. The results showed that the mRNAsi level of breast cancer was significantly higher than that of normal tissues (P=1.791e–43) (Figure 1A). The survival analysis showed that the low mRNAsi group had a higher overall survival rate than the high mRNAsi group. The 10-year survival rate of the mRNAsi group was about 57% with a 95% CI of (0.302–1.000), whereas the high mRNAsi group had a 10-year survival rate of about 22.4% with a 95% CI of (0.075–0.667) (P=0.046) (Figure 1B).
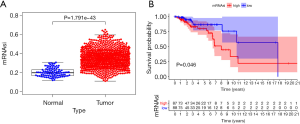
Differential gene expression analysis
In the TCGA data, a total of 3,830 differentially expressed genes were selected from 18,571 genes. The top 50 differential genes are shown in the heat map, in which the red indicates up-regulated genes and the green indicates down-regulated genes. It could be judged from the heat map that the expression levels of differentially expressed genes between normal tissues and cancer tissues were significantly different (Figure 2A). GEO data analysis showed that the gene expression level of African American women breast cancer tissue was similar to that of Mexican breast cancer tissue, with no statistical difference (|logFC| <1), but the gene expression level of African American women breast cancer tissue was significantly different from that of Mexican pericarcinomatous tissue. A total of 317 different genes were obtained according to the filtering criteria (Figure 2B). The differential genes expressed jointly by TCGA and GEO were obtained by making Venn diagram, a total of 218 co-expressed differential genes were obtained (Figure 2C).
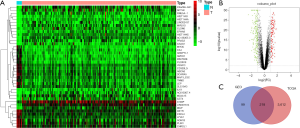
Co-expression analysis of WGCNA
The co-expression network of genes was constructed by WGCNA and the genes with similar functions were clustered for analysis. After identifying the modules with significant biological differences, the correlation between genes and breast cancer mRNAsi was analyzed (Figure 3A). Modules were clustered and the differential genes with variance <0.25 were analyzed. A total of three modules were constructed. The analysis results of module-trait relationship indicated that the correlations between the eight gene modules and mRNAsi were statistically different. In the positive correlation, the blue module was the most closely related to mRNAsi. In the negative correlation, blue and turquoise module were the most closely related to mRNAsi (Figure 3B). Among the three modules, all modules were not correlated with ereg-mRNAsi, the correlations with ereg-mRNAsi were not as significant as those with mRNAsi. According to the filtering standards cor. Gene MM >0.6 and cor. gene GS >0.5, 33 positively related key genes were selected from the blue module: KIF11, HMMR, SPC25, LMNB1, KIF20A, PBK, DEPDC1, ARHGAP11A, NUSAP1, BUB1, RAD51AP1, CEP55, KIF23, KIF4A, NEK2, AURKA, MELK, CCNA2, TPX2, FOXM1, GINS1, TTK, UBE2T, PTTG1, PLK1, CCNB2, NCAPG, KIF2C, CCNB1, NDC80, CKS2, EXO1, NUF2 (Figure 3C).
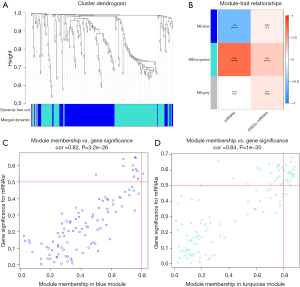
Key gene expression analysis
Key genes were differentially expressed between tumor tissues and cancer tissues and their expression levels in breast cancer tissues were significantly higher than those in normal tissues. The bar chart (Figure 4A) and heat map (Figure 4B) showed statistically significant differences between groups. The results showed that the correlation of most differentially expressed genes was greater than 0.5, which was consistent with the module cluster analysis (Figure 4C).
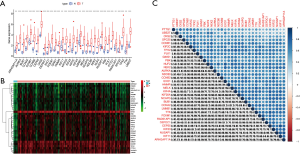
Protein interaction networks of hub genes
The protein interactions among key genes of 33 individuals were analyzed in STRING (https://www.string-db.org) version 11.0 (Figure 5A). KIF11, HMMR, SPC25, LMNB1, KIF20A, PBK, DEPDC1, ARHGAP11A, NUSAP1, BUB1, RAD51AP1, CEP55, KIF23, KIF4A, NEK2, AURKA, MELK, CCNA2, TPX2, FOXM1, GINS1, TTK, UBE2T, PTTG1, PLK1, CCNB2, NCAPG, KIF2C, CCNB1, NDC80, CKS2, EXO1, NUF2 were the most significant adjacent nodes in the network (Figure 5B). With the above six genes as the core of the interaction network, the data verification was conducted.
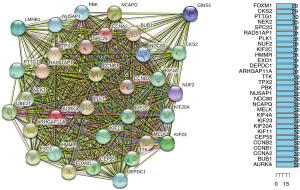
GO and KEGG enrichment analysis
The biological functions of key genes in the blue module was explored through GO analysis in three modes: BP, CC, and MF. Each model displayed the top 10 statistically significant functional groups. GO analysis suggested that the basic biological functions of the turquoise module included mitotic nuclear division, chromosome segregation, nuclear division, centromeric region and histone/serine/threonine/tyrosine kinase activity (Figure 6A). The KEGG analysis indicated that the biological functions of the turquoise module mainly involved cell cycle, progesterone-mediated oocyte maturation and oocyte meiosis (Figure 6B). Therefore, the functions of genes significantly associated with mRNAsi affected cell proliferation.
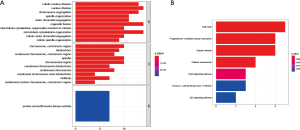
Differential expression of hub genes and sensitivity to chemotherapy drugs
The Stickecker Breast dataset from Oncomine was selected for Oncomine analysis. The dataset was included in Oncol Rep 2011/10/01. It contains 57 samples and involves 19,189 measurement genes. Three of the 33 key genes were selected for data validation. The expression levels of TTK, NCAPG, KIF2C in breast cancer tissues were higher than those in normal tissues. However, the expressions of key genes in triple-negative breast cancer tissues were higher than those in ERBB2-, ER- and PR-positive samples (Figure 7A). In addition, the response to epirubicin/cyclophosphamide + docetaxel in patients with the overexpression levels of TTK, NCAPG, KIF2C was better than that in the low expression group (Figure 7B).
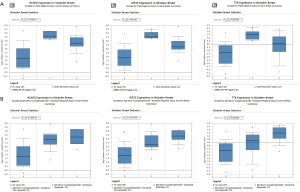
Discussion
Breast cancer is a highly heterogeneous and invasive disease. Tumor heterogeneity affects the target selection of drug therapy, weakens the therapeutic effect, and reduces patients' quality of life and survival rate. Compared with non-triple-negative breast cancer patients, triple-negative breast cancer patients showed the significantly decreased health status (10). Due to the lack of effective targets for endocrine therapy and targeted therapy in clinical practices (11), TNBC postoperative chemotherapy is still the main way to improve the survival rate in clinical practices (12,13), although the chemotherapy resistance exists (14). New study advances indicated that the high heterogeneity of breast cancer was ascribed to BCSCs and that the failure of chemotherapy and tumor recurrence and metastasis was ascribed to the proliferation and self-renewal abilities of BCSCs (15). Therefore, innovative treatment methods targeting BCSCs may be a new breakthrough point to improve the efficacy of breast cancer (16).
In this study, the key genes closely related to the characteristics of CSCs in WGCNA were screened based on mRNAsi. The higher mRNAsi correction score indicated that the stronger stem cell characteristics and the more significant dedifferentiation characteristics, which also suggested the lower overall survival of patients (17). The data analysis results showed that mRNAsi expression in tumor tissues was significantly higher than that in normal tissues. The key genes associated with mRNAsi were significantly up-regulated in cancer tissues, indicating that key genes played an important role in maintaining the characteristics of tumor stem cells and affected the formation, recurrence, metastasis and drug resistance of tumors (18).
According to the filtering criteria of GS and MM, the hub genes with similar functions were selected from the blue module. The selected genes were positively correlated with mRNAsi. Functional enrichment analysis suggested that the key genes were related to the self-renewal and proliferation characteristics of tumor stem cells. In the whole PPI network, TTK, NCAPG, KIF2C, and other genes had the most adjacent nodes. These genes were the key genes of the whole network. Oncomine meta-analysis also verified that the overexpression levels of these genes were closely related to the occurrence and development of invasive breast cancer. The high expression level of TTK was related to the mesenchymal and proliferative phenotypes of TNBC cells and the abnormal expression of TTK kinase was related to the occurrence, development and drug resistance in breast cancer and other cancers (19). NCAPG is mainly involved in the proliferation and metastasis of cancer cells (20,21). KIF2C is a prognostic indicator for a variety of tumors. KIF2C knockout can effectively inhibit the proliferation and metastasis of cancer cells (21). Therefore, TTK, NCAPG, KIF2C, and other genes were considered as hub genes of TNBC and might be the new targets for the treatment of TNBC.
Several studies showed that BCSCs had a variety of signaling pathways to regulate self-renewal and proliferation, such as Hedgehog, Notch, and PI3K/AKT/mTOR (22-24). In this study, GO and KEGG enrichment analysis revealed that the key genes not only affected the differentiation of BCSCs through the cell cycle and chromosome abnormalities, but also regulated the activity of histone kinase. BCSCs mainly exist in the G0 stage, when DNA synthesis or cell division does not occur. BCSCs do not enter the cell cycle unless the cell was stimulated. BCSCs enable damaged cells to have sufficient time for self-repair and achieve sustainable survival through DNA repair and anti-apoptotic ability (25). Cancer cells are characterized by multiple genomic defects, including extra chromosomes, chromosomal deletions, abnormal number of centrosomes (26). Loss of cancer-related gene heterozygosity in stem cells may result in the genetic instability of progeny cells and the occurrence of cancer (27). Abnormal chromosome separation during mitosis is a way for tumor cells to accumulate many abnormal genetic information needed for tumorigenesis (28). The mechanism of dynamic chromatin remodeling includes covalent histone modifications, histone variations, etc. Dynamic chromatin remodeling is the basis of many biological processes, including DNA replication and repair, chromosome condensation and separation, etc. Abnormalities in these processes are closely related to the development and progression of cancer (29). Epigenetic modifications of histones occur in key oncogenes, tumor suppressor genes and transcription factors, mediate gene expression and silencing, and regulate the plasticity of CSCs, and tumorigenesis (29,30).
Although TTK, NCAPG, KIF2C, and other genes were highly expressed in TNBC, compared with the breast cancer subtypes with the low expression of such genes, the highly expressed subtypes all responded better to docetaxel, suggesting that it was necessary to optimize the classification of TNBC subtypes for the clinical development of personalized chemotherapy (31). Keam found that TNBC with the high expression of Ki-67 was more responsive to docetaxel/doxorubicin and could obtain the better complete response rate (32). Lehmann found that different TNBC subtypes had significant differences in response to similar chemotherapy regimens (33). Therefore, the detection of the expression and proliferation of key genes is helpful for the preclinical prediction of the effect of chemotherapy.
In summary, 33 key genes were screened and determined to play an important role in maintaining the characteristics of BCSCs. The analysis of key genes is helpful to understand the characteristics of TNBC stem cells, optimize TNBC typing, guide the selection of chemotherapy regimens, and determine clinical outcomes. These genes are also potential therapeutic targets for the characteristics of BCSCs. The above conclusions should be further verified through biological experiments.
Acknowledgments
Funding: None.
Footnote
Data Sharing Statement: Available at http://dx.doi.org/10.21037/jxym-20-81
Conflicts of Interest: All authors have completed the ICMJE uniform disclosure form (available at http://dx.doi.org/10.21037/jxym-20-81). The authors have no conflicts of interest to declare.
Ethical Statement: The authors are accountable for all aspects of the work in ensuring that questions related to the accuracy or integrity of any part of the work are appropriately investigated and resolved. The study was conducted in accordance with the Declaration of Helsinki (as revised in 2013). All analyses were based on previous published data, thus no ethical approval and patient consent are required. The source of the data is clearly indicated in the article.
Open Access Statement: This is an Open Access article distributed in accordance with the Creative Commons Attribution-NonCommercial-NoDerivs 4.0 International License (CC BY-NC-ND 4.0), which permits the non-commercial replication and distribution of the article with the strict proviso that no changes or edits are made and the original work is properly cited (including links to both the formal publication through the relevant DOI and the license). See: https://creativecommons.org/licenses/by-nc-nd/4.0/.
References
- Davis Lynn BC, Rosenberg PS, Anderson WF, et al. Black-white breast cancer incidence trends: effects of ethnicity. J Natl Cancer Inst 2018;110:1270-2. [Crossref] [PubMed]
- Kelsey JL, Horn-Ross PL. Breast cancer: magnitude of the problem and descriptive epidemiology. Epidemiol Rev 1993;15:7-16. [Crossref] [PubMed]
- Koru-Sengul T, Santander AM, Miao F, et al. Breast cancers from black women exhibit higher numbers of immunosuppressive macrophages with proliferative activity and of crown-like structures associated with lower survival compared to non-black Latinas and Caucasians. Breast Cancer Res Treat 2016;158:113-26. [Crossref] [PubMed]
- Daly B, Olopade OI. Race, ethnicity, and the diagnosis of breast cancer. JAMA 2015;313:141-2. [Crossref] [PubMed]
- Grillet F, Bayet E, Villeronce O, et al. Circulating tumour cells from patients with colorectal cancer have cancer stem cell hallmarks in ex vivo culture. Gut 2017;66:1802-10. [Crossref] [PubMed]
- Chen DC, Liang YD, Peng L, et al. CBFA2T2 is associated with a cancer stem cell state in renal cell carcinoma. Cancer Cell Int 2017;17:103. [Crossref] [PubMed]
- Ram R, Brasch HD, Dunne JC, et al. The Identification of Three Cancer Stem Cell Subpopulations within Moderately Differentiated Lip Squamous Cell Carcinoma. Front Surg 2017;4:12. [Crossref] [PubMed]
- Kurimoto R, Takiguchi Y. Targeting epithelial-mesenchymal transition and cancer stem cell. In: Takiguchi Y. Molecular targeted therapy of lung cancer. Singapore: Springer, 2017:295-307.
- Gasch C, Ffrench B, O'Leary JJ, et al. Catching moving targets: cancer stem cell hierarchies, therapy-resistance & considerations for clinical intervention. Mol Cancer 2017;16:43. [Crossref] [PubMed]
- Vadaparampil ST, Christie J, Donovan KA, et al. Health-related quality of life in Black breast cancer survivors with and without triple-negative breast cancer (TNBC). Breast Cancer Res Treat 2017;163:331-42. [Crossref] [PubMed]
- Bhola NE, Jansen VM, Koch JP, et al. Treatment of triple-negative breast cancer with TORC1/2 inhibitors sustains a drug-resistant and notch-dependent cancer stem cell population. Cancer Res 2016;76:440-52. [Crossref] [PubMed]
- Zhang P, Yin Y, Mo H, et al. Better pathologic complete response and relapse-free survival after carboplatin plus paclitaxel compared with epirubicin plus paclitaxel as neoadjuvant chemotherapy for locally advanced triple-negative breast cancer: a randomized phase 2 trial. Oncotarget 2016;7:60647-56. [Crossref] [PubMed]
- Kim HA, Seong MK, Kim EK, et al. Evaluation of the survival benefit of different chemotherapy regimens in patients with T1-2N0 triple-negative breast cancer. J Breast Cancer 2015;18:271-8. [Crossref] [PubMed]
- Afghahi A, Timms KM, Vinayak S, et al. Tumor BRCA1 reversion mutation arising during neoadjuvant platinum-based chemotherapy in triple-negative breast cancer is associated with therapy resistance. Clin Cancer Res 2017;23:3365-70. [Crossref] [PubMed]
- Moreira MP, Brayner FA, Alves LC, et al. Phenotypic, structural, and ultrastructural analysis of triple-negative breast cancer cell lines and breast cancer stem cell subpopulation. Eur Biophys J 2019;48:673-84. [Crossref] [PubMed]
- Lee KL, Kuo YC, Ho YS, et al. Triple-negative breast cancer: current understanding and future therapeutic breakthrough targeting cancer stemness. Cancers (Basel) 2019;11:1334. [Crossref] [PubMed]
- Pan S, Zhan Y, Chen X, et al. Identification of biomarkers for controlling cancer stem cell characteristics in bladder cancer by network analysis of transcriptome data stemness indices. Front Oncol 2019;9:613. [Crossref] [PubMed]
- Dong L, Lyu X, Faleti OD, et al. The special stemness functions of Tbx3 in stem cells and cancer development. Semin Cancer Biol 2019;57:105-10. [Crossref] [PubMed]
- King JL, Zhang B, Li Y, et al. TTK promotes mesenchymal signaling via multiple mechanisms in triple negative breast cancer. Oncogenesis 2018;7:69. [Crossref] [PubMed]
- He Y, Tang J. Abstract P5-07-12: Circular RNA NCAPG promotes breast cancer metastasis through acting as the sponge of miR-200s. Cancer Res 2019; [Crossref]
- Zhang Q, Su R, Shan C, et al. Non-SMC condensin I complex, subunit G (NCAPG) is a novel mitotic gene required for hepatocellular cancer cell proliferation and migration. Oncol Res 2018;26:269-76. [Crossref] [PubMed]
- Zhou M, Hou Y, Yang G, et al. LncRNA-Hh strengthen cancer stem cells generation in twist-positive breast cancer via activation of hedgehog signaling pathway. Stem Cells 2016;34:55-66. [Crossref] [PubMed]
- Ray A, Vasudevan S, Sengupta S. 6-shogaol inhibits breast cancer cells and stem cell-like spheroids by modulation of notch signaling pathway and induction of autophagic cell death. PLoS One 2015;10:e0137614. [Crossref] [PubMed]
- Xia P, Xu XY. PI3K/Akt/mTOR signaling pathway in cancer stem cells: from basic research to clinical application. Am J Cancer Res 2015;5:1602-9. [PubMed]
- Shiotani B, Kobayashi M, Watanabe M, et al. Involvement of the ATR- and ATM-dependent checkpoint responses in cell cycle arrest evoked by pierisin-1. Mol Cancer Res 2006;4:125-33. [Crossref] [PubMed]
- Meraldi P, Honda R, Nigg EA. Aurora kinases link chromosome segregation and cell division to cancer susceptibility. Curr Opin Genet Dev 2004;14:29-36. [Crossref] [PubMed]
- Nguyen HG, Ravid K. Tetraploidy/aneuploidy and stem cells in cancer promotion: The role of chromosome passenger proteins. J Cell Physiol 2006;208:12-22. [Crossref] [PubMed]
- Gisselsson D. Classification of chromosome segregation errors in cancer. Chromosoma 2008;117:511-9. [Crossref] [PubMed]
- Wang GG, Allis CD, Chi P. Chromatin remodeling and cancer, Part I: covalent histone modifications. Trends Mol Med 2007;13:363-72. [Crossref] [PubMed]
- Shanmugam MK, Arfuso F, Arumugam S, et al. Correction: role of novel histone modifications in cancer. Oncotarget 2018;9:19460. [Crossref] [PubMed]
- Masuda H, Baggerly KA, Wang Y, et al. Differential response to neoadjuvant chemotherapy among 7 triple-negative breast cancer molecular subtypes. Clin Cancer Res 2013;19:5533-40. [Crossref] [PubMed]
- Keam B, Im SA, Lee KH, et al. Ki-67 can be used for further classification of triple negative breast cancer into two subtypes with different response and prognosis. Breast Cancer Res 2011;13:R22. [Crossref] [PubMed]
- Lehmann BD, Jovanović B, Chen X, et al. Refinement of triple-negative breast cancer molecular subtypes: implications for neoadjuvant chemotherapy selection. PLoS One 2016;11:e0157368. [Crossref] [PubMed]
Cite this article as: Zhang H, Qiu C, Zhu W. Identification of forward regulated hub genes and pathways for cancer stem cell characteristics in African American breast cancer by the network analysis. J Xiangya Med 2020;5:35.