Development of reference values and equations for the pulmonary function of Nigerian children aged 6–11 years measured with digital peak flow meter and its validation against local and the Global Lung Function Initiative (GLI) equations
Highlight box
Key findings
• Using an electronic peak flow meter (ePFM), Nigerian school-aged children achieved mean (standard deviation) peak expiratory flow (PEF) rate of 235.8 (52.0) L/min and forced expiratory volume in one second (FEV1) of 1.40 (0.34) L; both predicted mainly by height. While FEV1 fitted Global Lung Function Initiative (GLI)-African-American equations, PEF was mostly over-estimated by local and foreign equations.
What is known and what is new?
• Worldwide, there are several local and international reference standards for bench-marking lung-function values measured with mechanical peak flow meters and spirometers, but none for ePFM-measured lung-function.
• This is the first report of reference ePFM-measured PEF and FEV1, compared with published local/international equations.
What is the implication, and what should change now?
• GLI-African-American FEV1 equations can bench-mark ePFM-measured FEV1 of Nigerian children. However, published local and international PEF equations agreed poorly with our PEF; hence we develop new PEF equations for local use.
Introduction
Background
Spirometry is used to objectively diagnose and monitor chronic respiratory diseases (CRDs) such as asthma (1-3). Despite an enormous burden of CRDs, resource-constraint sub-Saharan countries like Nigeria suffer poor availability of spirometry services, especially for paediatric care, because of cost-, skill- and maintenance-related challenges (3-6). In such settings, mechanical peak flow meters (mPFM) such as mini-Wright PFM, which are cheaper and easier to use, have limited but valuable usefulness for lung function (LF) measurements (1,6,7). Thus, there are published local reference equations (REs) (8-12) for the peak expiratory flow (PEF) which is the maximum flow of air expired during a forced expiration after a maximal inspiration (2). While PEF can be used in the diagnosis and management of asthma (as percentage of personal best PEF, percentage PEF change from baseline or percentage of predicted PEF), it is highly effort-dependent potentially leading to large intra- and inter-person variability (1,13,14). In contrast, the forced expiratory volume in one second (FEV1), which is the volume of air that is expired forcefully by the first second of a maximal expiration after maximal inspiration, is a more reliable measure of LF, being less influenced by effort and diurnal variation (2,3,14). Fortunately, there are now digital or electronic peak flow meters (ePFM) which, unlike mPFM, measure both PEF and FEV1, and also have inbuilt quality checks and memory; these characteristics make ePFM more accurate than mPFMs (15-18).
Rationale and knowledge gap
Accurate interpretation of LF depends on the availability of REs that are most representative of a given population (2,13). The use of ePFMs in clinical settings may be limited by the non-availability of REs of PEF and FEV1 specifically measured with ePFM; REs derived from measurements made with mPFMs or spirometers may not be valid for LF measured with ePFMs because device-type affects the predictive accuracy of REs, potentially causing mis-diagnosis and over- or under-treatment (19,20). Although Arigliani et al. reported that the Global Lung Function Initiative (GLI)-African-American equations, including for FEV1, was valid for Nigerian children living in north-central Nigeria (21), this may not extrapolate to other parts of the country because of wide inter-regional socioeconomic, environmental and nutritional variations. There is thus need to further study the association of LF with these factors and anthropometric (measures of body size) or anthropomorphic factors (measures of body proportionality) in order to better account for LF variations within and between populations (22-26).
Objectives
Therefore, we (I) determined the mean and percentile values of ePFM-measured PEF and FEV1 of 6–11-year-old Nigerian children; (II) determined the association between LF and socio-demographic [age; sex; school type as a proxy for socioeconomic status (SES)], anthropometric [height, weight, lower limb length (LLL), chest circumference (CC)], anthropomorphic [body mass index (BMI), chest-circumference/height ratio (CC/H); upper-segment volume (UV)], nutritional (stunting, overweight/obesity) and environmental (diurnal variation, season) factors; (III) validated measured LF against Nigerian, non-Nigerian and GLI equations; and (IV) developed new PEF and FEV1 equations for Nigerian school-age children. We present this article in accordance with the reporting TRIPOD checklist for observational studies of the development and validation of prediction equations (available at https://jxym.amegroups.com/article/view/10.21037/jxym-23-29/rc) (27).
Methods
Design/population
This report is a secondary analysis of PEF and FEV1 data of healthy 6–11-year-old Nigerian school-children, originally enrolled from 14 primary schools into a cross-sectional study of their six-minute walk test (dataset: https://doi.org/10.17632/fcw8vvrxkw.1) (28). The procedural details of all anthropometric and lung-function measurements have been previously published (see supporting document at: https://doi.org/10.1002/ppul.25986) (29) and are summarized below.
Ethical approval and sample selection
The original study was conducted in accordance with the Declaration of Helsinki (as revised in 2013), and was approved by the Lagos State University Teaching Hospital (LASUTH)’s Health Research Ethics Committee (LREC/10/06/486). We also obtained written informed consent and assent from parents/guardians and the children, respectively. Using multi-stage random sampling, we enrolled healthy pupils from primary schools within Ikeja Local Government Area (LGA) of Lagos from 2016–2017. For this report, we excluded children with history/features suggestive of haematologic or cardiopulmonary disorders, recent respiratory tract infections (RTI) or those unable to perform LF maneuvers from our database of 943 children.
Data collection procedures
We used standard procedures (29) to measure weight with digital weight scale (OmronTM HN283, Omron Ltd., Japan), standing height with stadiometer (Prestige® Height Stand, Haerdik Medi-Tech, India), and both CC and LLL with an inelastic tape measure (inter-rater reliability for CC, LLL and height were respectively 0.997, 0.826 and 0.992) (29). We used a pocket-size ePFM (Asma-1™, Vitalograph, UK; https://vitalograph.com/product/asma-1-asthma-monitor/) which, according to the manufacturer, measures, and displays PEF in L/min and FEV1 in L using a stator rotor sensor. The device met the accuracy and precision standards of the American Thoracic Society (ATS)/European Respiratory Society (ERS) for spirometers [accuracy: better than ±3% (FEV1), ±10% (PEF); flow impedance: better than 0.15 kPa/L/s at 14 L/s; measurement range: FEV1: 0–9.99 L BTPS, PEF: 0–840 L/min BTPS]. Made for “clinic or personal home monitoring”, it has in-built quality check mechanism that flags and disqualifies measurements when there is any of: (I) ‘bad blows’ resulting from cough; (II) time to PEF >120 s; or (III) extrapolated volume >5% or >100 mL of FEV6. We conducted FEV1 maneuvers according to manufacturer’s instructions, in standing position, thus: with the head slightly elevated, the participant breathed in maximally and rapidly, placed the device with an attached disposable filter mouth-piece (SafeTwayTM, Vitalograph, Ireland) into the mouth maintaining a seal with the lips and immediately ‘blowed’ out the air “as hard and fast as possible”. These steps were repeated at least two more times. Nose-clips were not used. We recorded the best PEF and FEV1 of at least three attempts, using a single ePFM (Model No. 4,000; LOT No. 0107/2014) throughout the study (conducted mostly by P.O.U.). We did not conduct calibration checks on the device because, according to the manufacturer, it required no routine calibration; however, the lead author periodically used it to measure personal LF to ascertain consistent readings.
Data management
Statistical analyses were conducted with JASP Statistics version 0.18.0 (University of Amsterdam, the Netherlands) and Bluesky Statistics version 7.50 (BlueSky Statistics LLC, Chicago, USA), at significant P value of <0.05. We computed BMI [as weight in kg/(height in m)2]; CC/H; upper-segment length (UL) (as height minus LLL); upper-segment/height ratio (as US/H) and UV [as (CC2×US)/4π, assuming the chest to be a cylinder we used equation for cylinder volume; π=3.143] (30). The distribution of continuous variables was assessed with Shapiro-Wilk test and eye-balling of histograms. We used means [standard deviation (SD)] and frequencies to summarise continuous and categorical variables, respectively; and chi-square and student t-test (or ANOVA) to compare categorical and continuous variables among groups, respectively. We used Cohen’s d to quantify effect sizes of significant t-test comparisons: values <0.2, 0.2–0.49, 0.5–0.79 and 0.8 were graded trivial/negligible, small, moderate and large, respectively (31). We assessed linear correlation between LF and anthropometric and anthropomorphic variables with Pearson’s coefficient (r: <0.1, 0.3 and 0.5 implied trivial/small, moderate and strong correlations, respectively) (31). These correlations were also assessed with scatterplots with locally estimated scatterplot smoothing (LOESS) lines, rather than regression lines, to better assess the linearity of PEF and FEV1 across the range of the determinant variables. We used GLI’s online calculator (http://gli-calculator.ersnet.org/, GLI 2021, Version 2.0) to derive predicted FEV1 values and Z-scores (zFEV1) for our sample, and then tested the fit of our sample’s measured FEV1 to FEV1 predicted by the GLI equations (comprising four race-specific equations for African-Americans, Caucasians, South-east Asian and North-east Asian, and two race-neutral or race-composite equations, namely “Others” and the new “Global”) (23,32), using GLI’s validation criteria [mean (SD) predicted Z-score <0.5 (1.0) with 5% values below lower limits of normal (<1.645 Z-scores) and 5% above upper limit of normal (ULN) (>1.645 Z-scores)]. Furthermore, Cohen’s kappa (κ) was used to explore the agreement (concordance) of GLI-African American with each of GLI-Global and GLI-Others with respect to classification of participants with low FEV1 (zFEV1 <5th percentile or LLN). Since there are no GLI PEF equations, we calculated PEF Z-scores (zPEF) thus: (measured PEF − predicted PEF)/standard error of estimate (SEE) (33), with predicted PEF and SEE derived using previously published PEF equations for children in Lagos, Nigeria, by Faleti (34). Agreement between measured PEF and PEF predicted by Nigerian and non-Nigerian equations for school-age children was assessed with Bland-Altman analysis [mean bias and limits-of-agreement (LoAs) statistics] (35) based on two validation assumptions: (I) there is no significant mean bias (or error) between measured and predicted PEF when the 95% confidence intervals of the mean bias includes the line of equality (zero line) (36); (II) an acceptable LoA should not exceed 20% of the mean PEF for each sex, given that PEF may vary up to 20% between devices (3). We also developed new PEF and FEV1 prediction equations using stepwise multivariable linear regression models with outcome variables (OVs) being PEF or FEV1 and predictor variables being demo-anthropometric variables which show significant correlation with the OVs of at least small effect size (P<0.05; r≥0.2 or Cohen’s d ≥0.2) (37). Complete-case approach was adopted for all bivariate and multivariate analyses. We also used RefCurvTM (refcurv.com) (38), a free-source R-based software, to generate PEF and FEV1 smoothed percentile curves. RefCurvTM uses the General Additive Models for Location Scale and Shape (GAMLSS) add-on packages to generate penalized splines curves based on the Lamda-Mu-Sigma method using three parameters: L (skewness of distribution), M (median) and S (coefficient of variation). The best-fit smoothed percentile models were selected based on sets of hyperparameters (degrees of freedom) with the smallest Bayesian Information Criterion (BIC) (38).
Results
Out of 943 children in our database, 766 were eligible for this analysis (Figure 1).
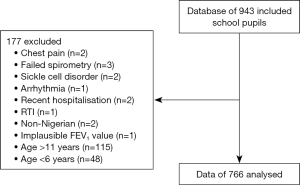
Characteristics of participants
These 766 children aged 6–11 years (53.1% girls) were from 14 primary schools (9 public) (Table 1). Girls and boys were similar in height, weight, and BMI, but girls were significantly smaller in most of the anthropomorphic variables, compared to boys: US/H [38.6% vs. 39.3%, P<0.001; Cohen’s d =−0.33], UV [15.8 vs. 16.6 L, P=0.014; Cohen’s d =−0.18], CC/H [0.48 vs. 0.49, P=0.042; Cohen’s d (95%) =−0.15], UL [49.8 vs. 50.8 cm, P<0.001; Cohen’s d =0.07] (Table 1).
Table 1
Characteristics | Girls (N=407) | Boys (N=359) | Total (N=766) | t† | P value† |
---|---|---|---|---|---|
Age, years | −0.081 | 0.26 | |||
Mean (SD) | 8.84 (1.58) | 8.97 (1.54) | 8.90 (1.56) | ||
Range | 6.00–11.90 | 6.00–11.80 | 6.00–11.90 | ||
Ethnic tribe, n (%) | 0.77‡ | 0.68 | |||
Yoruba | 194 (47.67) | 160 (44.57) | 354 (46.21) | ||
Igbo | 119 (29.24) | 113 (31.48) | 232 (30.29) | ||
Others | 94 (23.10) | 86 (23.96) | 180 (23.50) | ||
School category, n (%) | 0.725‡ | 0.39 | |||
Public | 220 (54.05) | 183 (50.97) | 403 (52.61) | ||
Private | 187 (45.95) | 176 (49.03) | 363 (47.39) | ||
Height, cm | −0.024 | 0.73 | |||
Mean (SD) | 129.23 (10.84) | 129.49 (10.46) | 129.35 (10.65) | ||
Range | 105.50–168.00 | 100.70–158.90 | 100.70–168.00 | ||
Weight, kg | −0.034 | 0.63 | |||
Mean (SD) | 30.29 (9.31) | 30.62 (10.19) | 30.44 (9.73) | ||
Range | 15.00–72.20 | 15.20–86.00 | 15.00–86.00 | ||
BMI, kg/m2 | −0.029 | 0.69 | |||
Mean (SD) | 17.76 (3.22) | 17.86 (3.51) | 17.81 (3.36) | ||
Range | 12.60–34.60 | 13.30–37.20 | 12.60–37.20 | ||
LLL, cm | 0.102 | 0.16 | |||
Mean (SD) | 79.44 (7.98) | 78.64 (7.76) | 79.06 (7.88) | ||
Range | 59.10–104.00 | 55.10–100.20 | 55.10–104.00 | ||
CC, cm | −0.128 | 0.07 | |||
Mean (SD) | 62.55 (6.52) | 63.43 (7.20) | 62.96 (6.85) | ||
Range | 48.20–90.30 | 50.20–101.30 | 48.20–101.30 | ||
UL, cm | −3.336 | <0.001 | |||
Mean (SD) | 49.79 (4.46) | 50.85 (4.32) | 50.29 (4.42) | ||
Range | 35.90–76.00 | 38.30–82.20 | 35.90–82.20 | ||
CC/H | −0.148 | 0.040 | |||
Mean (SD) | 0.48 (0.04) | 0.49 (0.04) | 0.49 (0.04) | ||
Range | 0.38–0.65 | 0.41–0.75 | 0.38–0.75 | ||
US/H, % | −4.540 | <0.001 | |||
Mean (SD) | 38.58 (2.27) | 39.32 (2.27) | 38.93 (2.30) | ||
Range | 30.55–54.32 | 33.54–59.87 | 30.55–59.87 | ||
UV, L | −2.475 | 0.01 | |||
Mean (SD) | 15.80 (4.31) | 16.65 (5.22) | 16.19 (4.77) | ||
Range | 7.93–33.52 | 8.14–47.68 | 7.93–47.68 | ||
WAZ | 0.064 | 0.45 | |||
Mean (SD) | 1.10 (0.87) | 1.04 (0.95) | 1.07 (0.90) | ||
Range | 0.00–5.04 | 0.00–5.40 | 0.00–5.40 | ||
HAZ | 0.039 | 0.59 | |||
Mean (SD) | 1.05 (0.76) | 1.02 (0.77) | 1.04 (0.77) | ||
Range | 0.00–3.70 | 0.00–4.00 | 0.00–4.00 | ||
BAZ | −0.137 | 0.05 | |||
Mean (SD) | 0.92 (0.86) | 1.05 (0.99) | 0.98 (0.92) | ||
Range | 0.00–4.90 | 0.00–5.80 | 0.00–5.80 | ||
PEF, L/min | −0.192 | 0.01 | |||
Mean (SD) | 231.06 (54.44) | 240.99 (48.67) | 235.76 (51.99) | ||
Range | 119.00–425.00 | 121.00–417.00 | 119.00–425.00 | ||
zPEF | 2.98 | 0.003 | |||
Mean (SD) | 0.138 (1.35) | −0.150 (1.17) | 0.002 (1.273) | ||
Range | −2.730 to 5.150 | −3.020 to 4.340 | −3.020 to 5.150 | ||
%predPEF, % | 2.66 | 0.008 | |||
Mean (SD) | 101.3 (14.98) | 98.43 (13.22) | 99.95 (14.23) | ||
Range | 62.87–161.54 | 71.27–151.07 | 62.87–161.54 | ||
FEV1, L | −0.24 | <0.001 | |||
Mean (SD) | 1.36 (0.34) | 1.44 (0.34) | 1.40 (0.34) | ||
Range | 0.61–2.80 | 0.65–2.59 | 0.61–2.80 | ||
zFEV1 | −2.51 | 0.01 | |||
Mean (SD) | −0.172 (1.104) | 0.028 (1.106) | −0.078 (1.109) | ||
Range | −3.280 to 4.060 | −3.330 to 3.430 | −3.330 to 4.060 | ||
%predFEV1, % | −2.654 | 0.008 | |||
Mean (SD) | 97.58 (14.55) | 100.38 (14.49) | 98.89 (14.58) | ||
Range | 55.98–150.61 | 57.60–142.19 | 55.98–150.61 |
†, independent Student t-test except otherwise stated; ‡, Pearson’s Chi-square test. Missing values: WAZ values were missing 108 girls and 106 boys; PEF values (and its derivatives—zPEF and %prePEF) values were missing for 52 girls and 40 boys. SD, standard deviation; BMI, body mass index; LLL, lower limb length, measured as distance from the anterior superior iliac spine to just below the medial malleolus of the right lower limb while standing; CC, chest circumference, measured at the level of the xiphisternum at maximum inspiration; UL, upper-segment length, derived as the difference between standing height and LLL; CC/H, chest-circumference/height ratio; US/H, upper-segment/height ratio, derived as the ratio of upper segment to standing height in percentage; UV, upper-segment volume, derived with the mathematical formula for the volume of a cylinder [(CC2 × UL)/4π, where π=3.143]; WAZ, weight-for-age Z-score derived with WHO’s Anthroplus® only for children aged 10 years and below; HAZ, height-for-age Z-score derived with WHO’s Anthroplus®; BAZ, BMI-for-age Z-score derived with WHO’s Anthroplus®; PEF, peak expiratory flow measured with Vitalograph®’s Asma-1 electronic peak flow meter; FEV1, forced expiratory volume in one second measured with Vitalograph®’s Asma-1 electronic peak flow meter; zPEF, Z-scores of PEF values derived with published PEF equations by Faleti [boys: PEF (L/min) = 8.447× age + 2.743× height – 186.166, R2=0.783, SEE =28.222; girls: PEF (L/min) =5.481× age + 2.733× height – 173.773, SEE =26.118, R2=0.761]; %predPEF, percentage of predicted FEV1 derived with Faleti equation; zFEV1, Z-scores of FEV1 values derived with GLI-2012 African-American equations; %predFEV1, percentage of predicted FEV1 derived with GLI-2012 equations.
Determinants of LF
Demographic factors and LF
The mean PEF was 231.1 [95% confidence interval (CI): 225.4–236.7] for girls, 241.0 (95% CI: 235.6–246.3) for boys and 235.8 (95% CI: 231.8–239.7) for the total sample, respectively, being significantly higher in boys [girls versus boys: P=0.013; Cohen’s d (95% CI): −0.19 (−0.34 to −0.04); Table 1]. The mean (95% CI) FEV1 was 1.36 (1.33–1.39) for girls, 1.44 (1.41–1.48) for boys and 1.40 (1.38–1.42) for the total sample, respectively, also significantly higher in boys [P<0.001; Cohen’s d (95% CI): −0.24 (−0.13 to −0.03)]. Although girls had significantly greater zPEF (PEF corrected for age and height) than boys, boys had greater zFEV1 (FEV1 corrected for age and height) than girls.
Table 2 shows age- and sex-based descriptive data including reference percentile values of both PEF and FEV1; both parameters increased linearly with age in boys and girls (P linear trend <0.001). Figures 2,3 show age- and sex-based PEF and FEV1 smoothed reference curves, respectively.
Table 2
Age | LF | Sex | N | Mean (SD) | Percentile values | ||||||
---|---|---|---|---|---|---|---|---|---|---|---|
5th | 10th | 25th | 50th | 75th | 90th | 95th | |||||
6 years | PEF (L/min) | Female | 54 | 181.1 (31.7) | 133.7 | 138.6 | 161 | 179 | 203 | 225.7 | 234.8 |
Male | 40 | 189.0 (27.4) | 148.1 | 159.8 | 172.8 | 193 | 210 | 222.4 | 227.4 | ||
FEV1 (L) | Female | 62 | 1.05 (0.20) | 0.72 | 0.78 | 0.9 | 1.05 | 1.19 | 1.32 | 1.38 | |
Male | 45 | 1.10 (0.21) | 0.78 | 0.81 | 0.97 | 1.11 | 1.2 | 1.34 | 1.41 | ||
7 years | PEF (L/min) | Female | 59 | 204.6 (34.1) | 155.7 | 157 | 186.5 | 204 | 229.5 | 242 | 261 |
Male | 42 | 207.6 (32.2) | 171.1 | 179 | 186 | 199 | 220.5 | 257.5 | 262.9 | ||
FEV1 (L) | Female | 64 | 1.22 (0.22) | 0.92 | 0.97 | 1.07 | 1.2 | 1.38 | 1.52 | 1.6 | |
Male | 50 | 1.20 (0.22) | 0.96 | 0.98 | 1.02 | 1.17 | 1.38 | 1.5 | 1.55 | ||
8 years | PEF (L/min) | Female | 73 | 215.5 (34.8) | 167.2 | 172.8 | 190 | 213 | 236 | 262 | 281.4 |
Male | 75 | 233.0 (38.6) | 177.8 | 185.8 | 210 | 228 | 250.5 | 278.6 | 297.1 | ||
FEV1 (L) | Female | 84 | 1.28 (0.25) | 1.01 | 1.04 | 1.11 | 1.23 | 1.42 | 1.67 | 1.74 | |
Male | 78 | 1.42 (0.21) | 1.14 | 1.17 | 1.29 | 1.42 | 1.54 | 1.66 | 1.79 | ||
9 years | PEF (L/min) | Female | 66 | 246.0 (51.9) | 186.5 | 192 | 213.3 | 230 | 270 | 324.5 | 343.3 |
Male | 64 | 253.0 (38.1) | 202.5 | 208.3 | 230 | 248.5 | 267.5 | 306.7 | 325 | ||
FEV1 (L) | Female | 80 | 1.44 (0.32) | 1 | 1.08 | 1.21 | 1.38 | 1.64 | 1.89 | 1.99 | |
Male | 74 | 1.53 (0.27) | 1.08 | 1.19 | 1.38 | 1.54 | 1.69 | 1.78 | 1.92 | ||
10 years | PEF (L/min) | Female | 69 | 265.6 (44.4) | 210 | 216 | 236 | 257 | 284 | 331.2 | 355.8 |
Male | 62 | 272.7 (46.7) | 216.3 | 225.2 | 242.3 | 259.5 | 296.5 | 336.7 | 367 | ||
FEV1 (L) | Female | 75 | 1.58 (0.28) | 1.19 | 1.26 | 1.36 | 1.57 | 1.77 | 1.94 | 2.03 | |
Male | 72 | 1.63 (0.36) | 1.18 | 1.22 | 1.37 | 1.6 | 1.87 | 2.1 | 2.19 | ||
11 years | PEF (L/min) | Female | 34 | 290.6 (60.3) | 221 | 225.8 | 240.5 | 278 | 329.8 | 364.9 | 416.5 |
Male | 36 | 278.3 (43.4) | 222.5 | 224.5 | 243 | 273.5 | 311 | 337 | 346.5 | ||
FEV1 (L) | Female | 42 | 1.68 (0.40) | 1.19 | 1.22 | 1.39 | 1.6 | 1.9 | 2.2 | 2.35 | |
Male | 40 | 1.71 (0.34) | 1.16 | 1.32 | 1.46 | 1.67 | 1.96 | 2.15 | 2.35 | ||
All | PEF† (L/min) | Female | 355 | 231.1 (54.4) | 156 | 166.8 | 192 | 224 | 260.5 | 302 | 338.6 |
Male | 319 | 241.0 (48.7) | 173 | 183.8 | 208.5 | 237 | 265.5 | 303 | 335.3 | ||
FEV1 (L) | Female | 407 | 1.36 (0.34) | 0.9 | 0.99 | 1.12 | 1.31 | 1.56 | 1.85 | 1.98 | |
Male | 359 | 1.45 (0.34) | 0.97 | 1.03 | 1.21 | 1.42 | 1.64 | 1.88 | 2.08 |
†, missing values: PEF values were missing for 52 girls and 40 boys. LF, lung function; N, number of participants; PEF, peak expiratory flow; FEV1, forced expiratory volume in one second; SD, standard deviation.
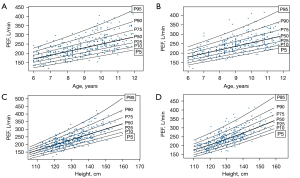
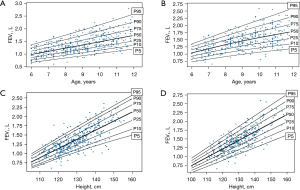
Linear correlation of LF with anthropometrics and anthropomorphics
In girls, boys and the total sample, each of PEF and FEV1 was strongly positively correlated with height (r=0.73–0.86), LLL (r=0.67–0.81), weight (r=0.62–0.76), age (r=0.60–0.66), UV (r=0.58–0.69), UL (r=0.54–0.61) and CC (r=0.52–0.66); generally, r was larger with FEV1 than PEF (Table 3). While the correlation of both PEF and FEV1 with BMI was moderate, their correlation with each of height-for-age Z-score (HAZ), weight-for-age Z-score (WAZ), BMI-for-age Z-score (BAZ), US/H and CC/H was weak or negligible. Figures 4,5 show the scatter-plots of these correlations in the total sample. Only age and height showed consistently linear trend with PEF and FEV1 across the ranges of their values (Figure 4A,4B,4G,4H); other variables showed varying degrees of non-linear relationship with PEF and FEV1 (Figure 4C-4F,4I,4J; Figure 5A-5J).
Table 3
Characteristics | All (n=674) | Girls (n=355) | Boys (n=319) | |||||
---|---|---|---|---|---|---|---|---|
PEF | FEV1 | PEF | FEV1 | PEF | FEV1 | |||
Height, cm | 0.74† | 0.78† | 0.76† | 0.86† | 0.73† | 0.81† | ||
LLL, cm | 0.69† | 0.72† | 0.72† | 0.81† | 0.67† | 0.75† | ||
Weight, kg | 0.63† | 0.69† | 0.65† | 0.76† | 0.62† | 0.75† | ||
Age, years | 0.63† | 0.60† | 0.64† | 0.66† | 0.62† | 0.64† | ||
UV, L | 0.58† | 0.66† | 0.62† | 0.68† | 0.55† | 0.64† | ||
UL, cm | 0.56† | 0.61† | 0.56† | 0.61† | 0.54† | 0.59† | ||
CC, cm | 0.53† | 0.62† | 0.55† | 0.66† | 0.52† | 0.66† | ||
BMI, kg/m2 | 0.39† | 0.46† | 0.38† | 0.47† | 0.41† | 0.55† | ||
US/H, % | −0.19† | −0.19† | −0.21† | −0.20† | −0.20† | −0.22† | ||
BAZ¶ | 0.17† | 0.23† | 0.12¶ | 0.19§ | 0.20§ | 0.31† | ||
WAZ | 0.17† | 0.18† | −0.16¶ | −0.10¶ | −0.09¶ | −0.12¶ | ||
HAZ | −0.01† | −0.11‡ | −0.04¶ | −0.11¶ | 0.04¶ | −0.07¶ | ||
CC/H | −0.06¶ | 0.03¶ | −0.11¶ | −0.08¶ | −0.01¶ | 0.09¶ |
†, P<0.001; ‡, P=0.002; §, P=0.01; ¶, P>0.05. PEF, peak expiratory flow, measured with VitalographTM’s Asma-1 electronic peak flow meter; FEV1, forced expiratory volume in one second, measured with VitalographTM’s Asma-1 electronic peak flow meter; LLL, lower limb length, measured as distance from the anterior superior iliac spine to just below the medial malleolus of the right lower limb while standing; UV, upper-segment volume, derived with equation for cylinder volume [(CC2 × UL)/4π, where CC is chest circumference measured at the level of the xiphisternum at maximum inspiration, UL is upper-segment length derived as the difference between standing height and LLL, and π=3.143]; US/H, upper-segment/height ratio, derived as the ratio of upper segment to standing height in percentage; BAZ, BMI-for-age Z-score derived with WHO’s Anthroplus®; WAZ, weight-for-age Z-score derived with WHO’s Anthroplus®; HAZ, height-for-age Z-score derived with WHO’s Anthroplus® derived with WHO’s Anthroplus®; CC/H, chest-circumference/height ratio; WAZ, weight-for-age Z-score derived with WHO’s BMI; BMI, body mass index; WHO, World Health Organization.
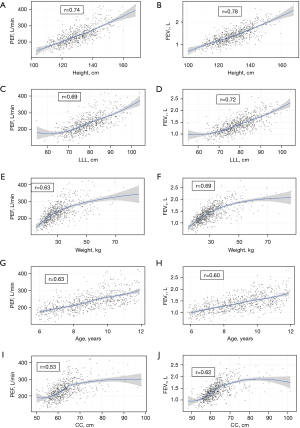
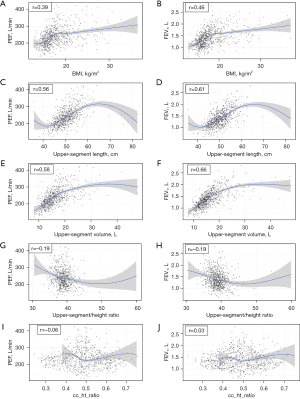
LF and socioeconomic, nutritional and environmental factors
Stunting did not affect LF [stunted vs. non-stunted: zPEF: 0.05 (1.17) vs. −0.003 (1.28), P=0.76); zFEV1: −0.19 (1.05) vs. −0.07 (1.11), P=0.37] (Table 4). Although overweight/obese and their normal-weight peers had similar zPEF [0.059 (1.444) vs. −0.022 (1.191), P=0.48], overweight/obese children had higher zFEV1 than their normal-weight counterparts [0.160 (1.127) vs. −0.171 (1.084), P<0.001, Cohen’s d =0.302]. Similarly, private school pupils had significantly higher FEV1 than public school peers (P<0.001). Diurnal variation did not influence either of PEF or FEV1, but PEF was significantly higher during the rainy season than during the dry season (Table 4).
Table 4
Factors | zPEF | zFEV1 | |||||||
---|---|---|---|---|---|---|---|---|---|
Mean (SD) | N | Statistic† | P | Mean | N | Statistic† | P | ||
Tribal ethnicity‡ | 0.765 | 0.47 | 1.52 | 0.22 | |||||
Igbo | 0.032 (1.189) | 207 | 0.01 (1.11) | 232 | |||||
Others | −0.106 (1.1) | 162 | −0.053 (1.013) | 180 | |||||
Yoruba | 0.038 (1.407) | 305 | −0.149 (1.151) | 354 | |||||
School type | 0.149 | 0.88 | 3.268 | 0.001 | |||||
Private | 0.009 (1.266) | 330 | 0.059 (1.093) | 363 | |||||
Public | −0.006 (1.281) | 344 | −0.202 (1.109) | 403 | |||||
Stunting§ | 0.299 | 0.76 | 0.887 | 0.37 | |||||
Stunted | 0.051 (1.173) | 54 | −0.194 (1.046) | 66 | |||||
Not stunted | −0.003 (1.282) | 620 | −0.06 (1.114) | 700 | |||||
Overweight/obesity¶ | −0.761 | 0.45 | 3.816 | <0.001 | |||||
Overweight/obese | 0.059 (1.444) | 206 | 0.16 (1.127) | 228 | |||||
Normal-weight | −0.022 (1.191) | 466 | −0.171 (1.084) | 534 | |||||
Diurnal period†† | 0.329 | 0.74 | −1.484 | 0.14 | |||||
AM | 0.061 (1.308) | 278 | −0.095 (1.047) | 309 | |||||
PM | 0.023 (1.324) | 271 | 0.036 (1.117) | 297 | |||||
Season‡‡ | −2.405 | 0.016 | −0.571 | 0.57 | |||||
Dry | −0.181 (1.065) | 198 | −0.114 (1.011) | 221 | |||||
Rainy | 0.077 (1.343) | 476 | −0.064 (1.146) | 545 |
†, statistic is chi-square value derived from a Pearson’s chi square analysis for categorical variables and t-value from an independent student t-test analysis for continuous variables; ‡, tribal ethnicity was based on parental self-report, usually paternal tribe; §, stunting was defined as height for age Z-score <−2 using WHO’s Anthroplus® version 1.0.4, WHO, Geneva 2009; ¶, overweight/obesity was defined as BMI Z-score <−1; ††, AM was morning period before and up till 12:00 noon while PM was afternoon period after 12:00 noon till about 2:30 pm; ‡‡, rainy season was approximately from April to November while dry season was from December to March. zPEF, Z-scores of PEF values derived with published PEF equations by Faleti (boys: PEF = 8.447 × age + 2.743 × height – 186.166, R2=0.783, SEE =28.222; girls: PEF = 5.481 × age + 2.733× height – 173.773, SEE =26.118, R2=0.761); %predPEF, percentage of predicted FEV1 derived with Faleti equation; zFEV1, Z-scores of FEV1 values derived with GLI-2012 African-American equations; %predFEV1, percentage of predicted FEV1 derived with GLI-2012 equations. PEF, peak expiratory flow; FEV1, forced expiratory volume in one second; SD, standard deviation; BMI, body mass index; GLI, Global Lung Function Initiative; WHO, World Health Organization; SEE, standard error of estimate.
Validation of measured PEF against local and foreign equations
The acceptable LoAs for girls and boys were ±46 L/min and ±48 L/min, respectively (20% of mean PEF for sex). Table 5 and Figure 6 shows that Nigerian and foreign PEF equations demonstrated significant mean biases from our measured PEF, except the equation by Faleti which showed the lower 95% boundary of the mean bias coincided approximately with the zero line. None of the equations showed LoAs within acceptable limits, including Faleti equation. We thus developed a new equation for the PEF of Nigerian children aged 6 to 11 years (Table 6).
Table 5
Author†† | Girls | Boys | |||||||
---|---|---|---|---|---|---|---|---|---|
PEF (%predPEF)† | Bias (95% CI)‡ | 95% LoA§ | P¶ | PEF (%predPEF)† | Bias (95% CI)‡ | 95% LoA§ | P¶ | ||
Faleti (34) (Nigeria) | 227.9±36.4 (101.3±15.0) | 3.61 (−0.07 to 7.28) | −59.0 to 79.0 | 0.054 | 245.2±38.7 (98.4±13.2) | −4.25 (−7.87 to −0.62) | −68.8 to 60.3 | 0.022 | |
Adeniyi (8) (Nigeria) | 212.0±36.9 (109.2±16.0) | 19.48 (15.81–23.15) | −49.4 to 88.4 | <0.001 | 213.5±35.6 (112.9±15.2) | 26.99 (23.38–30.60) | −37.2 to 91.2 | <0.001 | |
Agaba (9) (Nigeria) | 224.1±37.4 (103.2±15.6) | 7.38 (3.65–11.10) | −62.6 to 77.4 | <0.001 | 225.0±36.1 (107.0±14.7) | 15.33 (11.65–19.00) | −50.02 to 80.68 | <0.001 | |
Ahmed (12) (India) | 203.5±35.2 (99.2±41.3) | 27.95 (24.19–31.71) | −42.6 to 98.5 | <0.001 | 207.6±34.4 (103.1±39.5) | 32.72 (29.04–36.39) | −32.7 to 98.1 | <0.001 | |
Lu (11) (China) | 255.7±44.8 (79.0±32.9) | −24.11 (−27.85 to −20.38) | −94.2 to 46.0 | <0.001 | 268.0±45.9 (79.9±30.7) | −27.83 (−31.66 to −24.01) | −95.9 to 40.2 | <0.001 | |
Mittal (39) (India) | 210.4±41.2 (96.3±40.1) | 20.93 (17.21–24.65) | −49.0 to 90.8 | <0.001 | 211.9±44.6 (102.0±39.5) | 28.52 (−38.12 to −44.61) | −38.12 to 95.1 | <0.001 |
Missing values: PEF values were missing for 52 girls and 40 boys. †, data is mean ± SD of predicted PEF derived with respective PEF equations, with the mean ± SD of percentage of predicted PEF in bracket. ‡, mean bias (error) obtained from Bland-and-Altman analyses. §, 95% LoA (lower and upper limits) from Bland-and-Altman analyses. Acceptable 95% LoA was defined as ±46 and ±48 L/min for girls and boys, respectively (20% of mean PEF for girls and boys). ¶, P values for a paired t-test comparison between measured PEF and PEF predicted by the corresponding equations. ††, all the studies measured PEF with manual peak flow meters except the Lagos-Nigeria study by Faleti that used a hand-held portable digital spirometer (OneFlowTM). PEF, peak expiratory flow, measured with VitalographTM’s Asma-1 electronic peak flow meter; %predPEF, percentage of predicted FEV1 derived with Faleti equation; CI, confidence interval; LoA, limit of agreement; SD, standard deviation; FEV1, forced expiratory volume in one second.
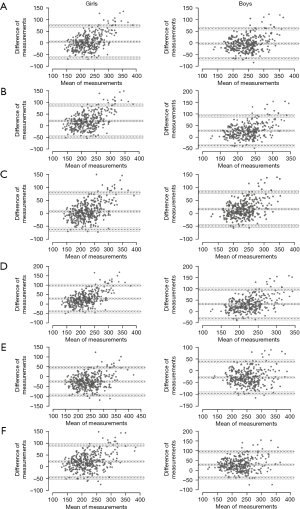
Table 6
Equations | Adjusted R2 | SEE |
---|---|---|
†PEF = 6.4 × (ageyears) + 2.95 × (heightcm) − 202.9 | 56.9 | 34.13 |
‡FEV1 = 0.025 × (heightcm) − 1.836 | 61.0 | 0.213 |
†, variables added include height, LLL, weight, age, sex, upper segment volume, BMI, upper segment length, chest circumference. Of these, only four variable were automatically retained in an initial model: height, age, weight and sex. However, the contributions of sex and weight to the model were negligible being <0.01%, and so both were deleted to yield the final model. Age added only 1.7%; N=674. Max variable inflation factor was 2.1. ‡, variables added include height, LLL, weight, age, sex, upper segment volume, BMI, upper segment length, chest circumference. Of these, chest circumference, sex and age contributed 1.6%, 1.0% and 0.4%, respectively, and so were deleted to yield a simpler final model consisting of height as the only predictor; N=766. An example of the clinical application of the equations: to determine the predicted FEV1 of a 10-year-old girl who is 120 cm tall with measured FEV1 of 1.3 L, we use the equation FEV1 = 0.025 (Heightcm) – 1.836, thus: (0.025×120)−1.836=1.16 L. The percentage predicted FEV1 is (1.3/1.16)×100=112%. To calculate the FEV1 Z-score, we use the formula (observed FEV1 − predicted FEV1)/SEE = (1.3−1.16)/0.213=0.66. Table 2 shows that FEV1 of 1.30 L falls between the 10th and 25th percentiles. PEF, peak expiratory flow; SEE, standard error of estimate; FEV1, forced expiratory volume in one second; LLL, lower limb length; BMI, body mass index.
Validation of FEV1 against GLI equations
Table 7 shows that, out of the six GLI equations, only the African-American (for boys and girls) and the south-east Asian equations (for girls) fitted our FEV1; other GLI equations significantly over-estimated our sample’s FEV1. With respect to classification of participants with zFEV1 < LLN, GLI-African-American equations had slightly better agreement with GLI-Others [κ (95% CI): 0.50 (0.42–0.58)] than with GLI-Global [κ (95% CI): 0.48 (0.40–0.56)].
Table 7
GLI-equation | Sex | Predicted zFEV1 | Limits of normal | |||
---|---|---|---|---|---|---|
Mean (SD) | Range | LLN, n (%) | ULN, n (%) | |||
African-American | Female | −0.17 (1.10) | −3.28 to 4.06 | 37 (9.09) | 22 (5.40) | |
Male | 0.03 (1.11) | −3.33 to 3.43 | 26 (7.24) | 27 (7.52) | ||
All | −0.08 (1.11) | −3.33 to 4.06 | 63 (8.22) | 49 (6.40) | ||
Caucasian | Female | −1.31 (1.03) | −4.23 to 2.62 | 319 (78.38) | 2 (0.49) | |
Male | −1.22 (1.05) | −4.43 to 1.92 | 265 (73.82) | 1 (0.28) | ||
All | −1.27 (1.35) | −4.43 to 2.62 | 584 (76.24) | 3 (0.39) | ||
NE Asian | Female | −1.22 (1.06) | −4.22 to 2.82 | 131 (32.19) | 3 (0.74) | |
Male | −1.43 (1.62) | −6.37 to 3.44 | 154 (42.90) | 14 (3.90) | ||
All | −1.32 (1.35) | −6.37 to 3.44 | 285 (37.21) | 17 (2.22) | ||
SE Asian | Female | −0.39 (1.10) | −3.49 to 3.83 | 50 (12.28) | 16 (3.93) | |
Male | −0.54 (1.11) | −3.92 to 2.84 | 52 (14.48) | 11 (3.06) | ||
All | −0.46 (1.11) | −3.92 to 3.83 | 102 (13.32) | 27 (3.52) | ||
Others/mixed | Female | −0.79 (1.11) | −3.92 to 3.43 | 80 (19.66) | 8 (1.97) | |
Male | −0.68 (1.11) | −4.08 to 2.70 | 64 (17.83) | 9 (2.51) | ||
All | −0.74 (1.11) | −4.08 to 3.43 | 144 (18.80) | 17 (2.23) | ||
Global (race-neutral) | Female | −1.04 (0.97) | −3.71 to 3.19 | 98 (24.1) | 2 (0.5) | |
Male | −0.87 (0.97) | −3.56 to 1.99 | 17 (19.8) | 6 (1.7) | ||
All | −0.96 (0.98) | −3.71 to 3.19 | 169 (22.06) | 8 (1.04) |
N is 407 and 359 for girls and boys, respectively. In girls, the LLN (5th percentile) for GLI-African-American equation corresponded to <1.956 and −1.675 for FEV1 and PEF respectively, while it corresponded to <−1.790 and −1.702 for FEV1 and PEF, respectively, in boys. †, fit to GLI-2012 equations was defined as mean (SD) Z-scores of <0.5 with SD of about 1 with 5% of Z-scores below −1.64 (LLN) and 5% above +1.64 (ULN). GLI, Global Lung Function Initiative; FEV1, forced expiratory volume in one second; zFEV1, Z-scores of FEV1 values derived with respective GLI equations; LLN, proportion of Z-score values below the 5th percentile; ULN, proportion of values above the 95th percentile; NE, north-eastern; SE, south-eastern; PEF, peak expiratory flow; SD, standard deviation.
Discussion
Key findings
The ePFM-measured mean PEF and FEV1 of Nigerian school-aged children were 235.8 L/min and 1.40 L, respectively, with height being the most influential determinant. Previously published Nigerian and foreign PEF equations agreed poorly with our sample’s PEF due to their wide LoA and significant bias, although PEF equations of Nigerian children in Lagos derived by Faleti (34) showed small mean bias. For FEV1, only the GLI-African-American equations fitted both boys and girls; other equations, including the new ‘race-neutral’ GLI-Global (23,24) recently recommended by the ATS for global benchmarking of LF, over-estimated the FEV1. Our study is one of the first few to explore the applicability of the new GLI-Global equations, especially in African paediatric populations (40,41).
Strengths and limitations
We had a large sample size, resulting in our narrow confidence intervals; this strengthens the precision of our estimates, internal validity and generatability, despite some missing PEF data. Because ePFM does not measure forced vital capacity (FVC), we could not provide data on FEV1/FVC ratio which is a more precise indicator of airway obstruction than FEV1 alone (13). However, we aimed specifically to provide ePFM-measured reference values for PEF and FEV1 for pulmonary evaluation and care in low-resource settings, in the absence of standard spirometers. Also, the device does not have graphical displays for quality assurance (flow-volume or flow-time curves); however, its inbuilt quality checks make ePFM superior to mPFM (13). We studied a narrow age-range (6–11 years) of Nigerian children, implying that our findings and equations may not apply to other paediatric sub-groups. There may be the risk of stitching our equations with others—a practice known to result in misinterpretations (32). Other determinants of LF like maternal smoking, prematurity, low birth-weight, second-hand smoking and exposure to air-pollution were not evaluated or accounted for in our study.
ePFM versus mPFM
The previously highlighted characteristics of ePFM make them superior to mPFM for home self-monitoring of LF and for clinical diagnosis and research. For example, in asthma diagnosis, FEV1-based bronchodilator reversibility tests conducted with ePFM are preferred to PEF-based tests done with mPFM (1,3). Also, the in-built memory in ePFM allow clinicians, in the hospital, to retrieve and review the patient’s home-measured PEF and FEV1 from the device, or even remotely, precluding the need for paper-based recording of home measurements (18,42,43). Additionally, patients are unable to falsify LF readings made with ePFM, as may occur with paper-based recordings when using mPFM (17).
Comparison with similar researches and clinical implications
Mean PEF and FEV1 values of Nigerian children
Online searches did not yield similar study of ePFM-measured LF for comparison; hence, we compared our measurements with those measured with spirometers or mPFMs, cautiously. Of note was that our sample’s mean PEF (235.8 L/min) and FEV1 values (1.40 L) were strikingly similar to PEF of 230.3 L/min and FEV1 of 1.48 L reported by Faleti et al. (34,44) among 5–12-year-old children in the same locality of Ikeja, Lagos, Nigeria, using an hand-held digital spirometer (One FlowTM) [2011–2012]. This perhaps suggests that ePFMs have accuracy similar to spirometers, as previously reported in studies that compared both device types (15,16). When our findings are compared with studies that used mini-Wright mPFM among Nigerian children, our mean PEF exceeded 212 L/min observed in Jos plateau (Northern Nigeria) by Agaba et al. (9) and 216 L/min reported in Abuja (North-central Nigeria) by Adeniyi (8). It was however lower than 257 L/min reported in Kano (Northern Nigeria) by Mohammed et al. (45). These differences may be due to differences in LF device-types, inclusion/exclusion criteria, methodology, study population, ethno-geographic locations or socio-economic status.
Agreement of PEF of Nigerian children with published PEF equations
In asthma management, it is most preferred to compare a person’s LF values with his or her personal best values (3), but this is often not possible because it requires consistent measurements at home for 2 weeks while symptom-free. Thus, normative LF references are still needed for benchmarking measured LF (13). Determining which equation is representative of a given population entails appropriate statistical comparisons with measured values from healthy subjects. Rather than use bivariate statistical tests like t-test to compare measured and predicted PEF values as was done by some authors (10), we used the Bland-Altman analysis to assess true agreement between them; t-test or even linear correlation tests do not provide valid assessments of agreement between variables (35). The significant mean bias (except for Faleti’s equations) and wide LoAs observed with all the PEF equations suggests caution in the use of these equations for our population. Although comparison of our measured PEF with PEF predicted with Faleti’s equation yielded small bias and non-significant paired t-test P value (Table 5), the limit-of-agreement was wide, implying unsatisfactory agreement. This may be due to the use of a relatively small sample size of 100 children Faleti’s study which may have increased the statistical variability of their estimates.
Fit of FEV1 of Nigerian children to GLI-2012 equations
Recently, ATS (24) recommended the evaluation and use of newly developed race-neutral GLI-Global, over race-specific GLI equations. However, researchers and clinicians, especially in populations not included in the original GLI data, still need to decide which GLI equations are most applicable to their populations (46). Thus, we tested our FEV1 against GLI FEV1 equations (32). Masekela et al. (19), in a systematic review of studies that evaluated GLI equations in seven African countries (namely, Algeria, Angola, Benin, Congo, Tunisia, Madagascar, South-Africa) up till November 2018, reported that GLI-African-American equations were valid for sub-Saharan populations, except West Africans. However, the latter was represented only by Beninese children, which may not apply to Nigerian children. Subsequently, Arigliani et al. (21) in a study of Nigerian children and adolescents in northern Nigeria, reported that the GLI-African-American equation fitted them. Similarly, our sample’s FEV1 fitted the GLI-African-American equation [−0.08 (1.11)], even better than that reported by Arigliani et al. (21) [−0.35 (0.99)]. Compared to adolescence and adulthood, GLI equations appear to fit pre-adolescent age-groups (like our sample) better than adolescent and adult age-groups (47,48), perhaps because the effect of underlying and ongoing determinants of LF become more apparent as the individual grows from childhood to adolescence/adulthood. Thus, whereas GLI-African-American equations fit Nigerian children and adolescents, Fawibe et al. (49) reported that it did not fit Nigerian adult (however the latter study did not use GLI’s validation criteria to determine true fit). Other authors from other African countries have reported that the GLI-African-American FEV1 equations to fit Zimbabwean (50), Cameroonian (51), Congolese, Madagascan, and Angolan children (52) but not South-African black children (53).
Race-neutral vs. race-based GLI FEV1 equations
Race-based GLI equations (including GLI-African-American) may reflect and perpetuate structural racism and socioeconomic and health inequities, rather than reflecting true racial differences (23,24). Yet, certain observations still suggests the influence of race, over socioeconomic factors, on LF: despite the fact that African-American persons, and not indigenous Africans, were included in the GLI dataset and that both groups grew in varied environmental and socioeconomic conditions, the GLI-African-American equations fits the FEV1 of indigenous Nigerian children (21). Similarly, indigenous Nigerian children demonstrated FEV1 similar to Nigerian children born and living in the UK (54). Contrastingly, the zFEV1 of indigenous Indian children was significantly lower than that of UK-resident counterparts; however, the zFEV1 was similar between urban-dwelling indigenous Indian children and their UK-resident peers, while it was lower in rural-dwelling indigenous ones than UK-resident Indian children (55). This suggests that socioeconomic conditions also affect LF and development, additional to racial influence.
The GLI-Others and GLI-Global equations are ‘race-composite’ having been derived as combination of GLI race-based equations; the new GLI-Global equation is a ‘weighted’ derivative of the race-based equations, so as to balance out the effect of differences in the proportion of data contributed into the global dataset from different regions (23). We explored potential impact of these two equations on clinical diagnosis of low FEV1 (zFEV1 < LLN). GLI-Others and GLI-Global categorised 19% and 22%, respectively, of our sample as low zFEV1 while GLI-African-American identified 10% as low zFEV1. Whether the race-composite equations (GLI-Others and GLI-Global) over-diagnose low LF or that GLI-African-American equations under-diagnose it among our population requires further comparison of their association with clinical outcomes among diseased groups (23,24,40,41). However, our test of concordance suggests that the agreement between GLI-Global and GLI-African-American equations was slightly less than between GLI-African-American and GLI-Others.
Anthro-demographic and anthropomorphic correlation with LF
Well-known determinants of childhood LF include standing height, sitting height, age, weight, CC, LLL and chest volume. In agreement with previous authors (10-12,22,34,39,56-59), standing height showed strongest correlation with PEF and FEV1. Additionally, two surrogate measures of thoracic volume—UV and UL—also showed correlation with LF, albeit of less magnitude than height. That height, compared to other variables, had the largest correlation with LF (in both bivariate and multivariable analyses) suggests it is a better composite measure of thoracic volume, inspiratory muscle strength, lung distensibility and other less-measurable LF determinants. However, there is still need for variables that may predict LF better than height; few studies suggest that ulna length and arm-span may (46,56,60).
Socio-demographic and environmental determinants of LF
Poverty is associated with stunting which in turn predisposes to lower LF due to smaller lung volume (54). However, we found that stunted and non-stunted children had similar LF in our urban sample. Also, in contrast to Madanhire et al.’s findings among Zimbabwean children (50), LF was affected by school type (a measure of SES) in our study, possibly because children in private-schools were heavier than those in public-schools (data not shown) and we observed that heavier children had FEV1 that was higher than normal-weight children [similarly observed among children in northern-Nigeria by Mohammed et al. (45)]. However, the higher mean FEV1 among overweight/obese children may not imply that they have better LF since we did not measure FVC which is required to determine FEV1/FVC ratio. Due to asymmetric lung growth (dysanapsis), overweight/obese children often have FEV1 and FVC values that are higher than normal-weight peers, but the FVC is often disproportionately higher than the FEV1 resulting in reduced FEV1/FVC (61,62). Like many authors (8,9,11,39,50,63,64), but in contrast to others (45,58), we observed significant sex differences in PEF and FEV1. The lower FEV1 noted among girls may be due to their smaller thoracic cavity. African girls, compared to boys, are more exposed to household-air pollutants arising from cooking and cleaning activities, and consequent impaired lung growth (65).
Clinical application of prediction PEF and FEV1 equations
Simple linear regression equations like ours can estimate the lower-limit-of-normal, percentage of predicted and Z-score values of PEF and FEV1 measurements (see Table 6 and its footnote) (33). Whereas prediction models derived with advanced statistical tools like GAMLSS account better for LF variability along a wider age range, their differences from linear regression equations may be clinically inconsequential (66), especially when dealing with a narrow age-range such as the school-age group when there is predominantly a linear relationship between height and LF (32). Height-based equations may be more clinically useful than age- or weight-based reference standards in low-resource settings because height is less affected by acute illnesses (like weight), and it is not affected by recall bias (like age).
Conclusions
The GLI-African-American FEV1 equations are valid for bench-marking FEV1 of school-aged Nigerian children measured with ePFM especially when there are no spirometers. However, our sample’s PEF did not agree acceptably with published local and foreign PEF equations. Thus, we developed new prediction PEF and FEV1 (as well as reference curves) for possible clinical use after further external validation in separate local samples. Multi-centre nation-wide studies of the LF of the Nigerian population spanning childhood to adulthood (with the use of cutting-edge advanced statistical tools like GAMLSS) across varied socioeconomic and geographical characteristics are needed for optimal respiratory care. These and those from other sub-Saharan African populations are needed to update the GLI database (67). Additionally, the relative accuracy of GLI-Global, GLI-Others and GLI-African-American equations, and local-derived equations, in predicting relevant clinical outcomes needs further exploration.
Acknowledgments
We express heartfelt gratitude to the school pupils and their parents/guardians, as well as to the Lagos State Universal Basic Education Board (SUBEB), the Lagos State Ministry of Education, school headteachers, proprietors and school staff members for providing support for the study. We also thank Engineer Chris’ Ubuane for helping to procure the peak flow meter and mouthpieces from the UK, and also the research assistants. We also thank Dr Christian Winkler for enormously supporting the use of RefCurvTM, especially for providing additional capabilities for the 5th and 95th centile curves.
Parts of these results were published as conference abstracts of the Nigerian Thoracic Society’s Annual Scientific Conference 2022 and the Pan African Thoracic Society/Respiratory Society of Kenya Congress 2023.
Funding: None.
Footnote
Reporting Checklist: The authors have completed the TRIPOD reporting checklist. Available at https://jxym.amegroups.com/article/view/10.21037/jxym-23-29/rc
Data Sharing Statement: Available at https://jxym.amegroups.com/article/view/10.21037/jxym-23-29/dss
Peer Review File: Available at https://jxym.amegroups.com/article/view/10.21037/jxym-23-29/prf
Conflicts of Interest: All authors have completed the ICMJE uniform disclosure form (available at https://jxym.amegroups.com/article/view/10.21037/jxym-23-29/coif). The authors have no conflicts of interest to declare.
Ethical Statement: The authors are accountable for all aspects of the work in ensuring that questions related to the accuracy or integrity of any part of the work are appropriately investigated and resolved. The study was conducted in accordance with the Declaration of Helsinki (as revised in 2013). The study was approved by the Health Research Ethics Committee of the Lagos State University Teaching Hospital (LASUTH), Ikeja, Lagos, Nigeria (LREC/10/06/486), and we obtained written informed consent and assent from parents/guardians and their children, respectively, prior to enrolment.
Open Access Statement: This is an Open Access article distributed in accordance with the Creative Commons Attribution-NonCommercial-NoDerivs 4.0 International License (CC BY-NC-ND 4.0), which permits the non-commercial replication and distribution of the article with the strict proviso that no changes or edits are made and the original work is properly cited (including links to both the formal publication through the relevant DOI and the license). See: https://creativecommons.org/licenses/by-nc-nd/4.0/.
References
- Gaillard EA, Kuehni CE, Turner S, et al. European Respiratory Society clinical practice guidelines for the diagnosis of asthma in children aged 5-16 years. Eur Respir J 2021;58:2004173. [Crossref] [PubMed]
- Graham BL, Steenbruggen I, Miller MR, et al. Standardization of Spirometry 2019 Update. An Official American Thoracic Society and European Respiratory Society Technical Statement. Am J Respir Crit Care Med 2019;200:e70-88. [Crossref] [PubMed]
- Global Initiative for Asthma (GINA). Global strategy for asthma management and prevention, updated 2023. 2023.
- Masekela R, Zurba L, Gray D. Dealing with Access to Spirometry in Africa: A Commentary on Challenges and Solutions. Int J Environ Res Public Health 2018;16:62. [Crossref] [PubMed]
- Plum C, Stolbrink M, Zurba L, et al. Availability of diagnostic services and essential medicines for non-communicable respiratory diseases in African countries. Int J Tuberc Lung Dis 2021;25:120-5. [Crossref] [PubMed]
- Mortimer K, Masekela R, Ozoh OB, et al. The reality of managing asthma in sub-Saharan Africa – Priorities and strategies for improving care. J Pan Afr Thorac Soc 2022;3:105-20. [Crossref]
- Ojo O, Ajibare A, Odeyemi A, Fapohunda T, Adeyeye O. Clinical utility of peak flow meter in asthma diagnosis and monitoring in low- and middle-income countries: A narrative review. Int J Med Heal Dev 2023;28:87. [Crossref]
- Adeniyi FB. Peak expiratory flow rate in healthy urban Nigerian school children in Abuja, Nigeria. Stellenbosch University, 2018.
- Agaba PA, Thacher TD, Angyo IA, et al. Peak expiratory flow rates in healthy Nigerian children. J Trop Pediatr 2003;49:157-9. [Crossref] [PubMed]
- Mojiminiyi FBO, Igbokwe UV, Ajagbonna OP, et al. Peak expiratory flow rate in normal Hausa-Fulani children and adolescents of Northern Nigeria. Ann Afr Med 2006;5:10-5.
- Lu Y, Zheng J, Liu C, et al. Peak expiratory flow among healthy children aged 5-14 years in China. J Thorac Dis 2018;10:1377-85. [Crossref] [PubMed]
- Ahmed M, Gavhane J, Goel S. Age Specific Peak Expiratory Flow Nomogram in Children. Indian J Pediatr 2017;84:271-5. [Crossref] [PubMed]
- Stanojevic S, Kaminsky DA, Miller MR, et al. ERS/ATS technical standard on interpretive strategies for routine lung function tests. Eur Respir J 2022;60:2101499. [Crossref] [PubMed]
- Miller MR, Hankinson J, Brusasco V, et al. ATS/ERS task force. Standardisation of spirometry. Eur Respir J 2005;26:319-38. [Crossref] [PubMed]
- do Nascimento Maia P, Bazhuni Pombo Sant'Anna MF, Parente AAAI, et al. Correlation of digital flow peak with spirometry in children with and without asthma. J Asthma 2023;60:270-6. [Crossref] [PubMed]
- Aguilar-Fernández AJ, Villa-Asensi JR, Castro-Codesal M, et al. Concordance between the Piko - 1 portable device and pneumotachography in measuring PEF and FEV(1) in asthmatic children. Allergol Immunopathol (Madr) 2009;37:244-8. [Crossref] [PubMed]
- Kamps AW, Roorda RJ, Brand PL. Peak flow diaries in childhood asthma are unreliable. Thorax 2001;56:180-2. [Crossref] [PubMed]
- Sakkatos P, Williams A. Testing the accuracy of a novel digital peak flow meter aligned with a smartphone app compared to a lab spirometer: A pilot work. Digit Health 2021;7:20552076211005959. [Crossref] [PubMed]
- Masekela R, Hall GL, Stanojevic S, et al. An urgent need for African spirometry reference equations: the Paediatric and Adult African Spirometry study. Int J Tuberc Lung Dis 2019;23:952-8. [Crossref] [PubMed]
- Milanzi EB, Koppelman GH, Oldenwening M, et al. Considerations in the use of different spirometers in epidemiological studies. Environ Health 2019;18:39. [Crossref] [PubMed]
- Arigliani M, Castriotta L, Zubair R, et al. Differences in lung function between children with sickle cell anaemia from West Africa and Europe. Thorax 2019;74:1154-60. [Crossref] [PubMed]
- Lum S, Bountziouka V, Sonnappa S, et al. Lung function in children in relation to ethnicity, physique and socioeconomic factors. Eur Respir J 2015;46:1662-71. [Crossref] [PubMed]
- Bowerman C, Bhakta NR, Brazzale D, et al. A Race-neutral Approach to the Interpretation of Lung Function Measurements. Am J Respir Crit Care Med 2023;207:768-74. [Crossref] [PubMed]
- Bhakta NR, Bime C, Kaminsky DA, et al. Race and Ethnicity in Pulmonary Function Test Interpretation: An Official American Thoracic Society Statement. Am J Respir Crit Care Med 2023;207:978-95. [Crossref] [PubMed]
- Bhakta NR, Kaminsky DA, Bime C, et al. Addressing Race in Pulmonary Function Testing by Aligning Intent and Evidence With Practice and Perception. Chest 2022;161:288-97. [Crossref] [PubMed]
- Holland R, Bowerman C, Stanojevic S. The Contribution of Anthropometry and Socioeconomic Status to Racial Differences in Measures of Lung Function: A Systematic Review. Chest 2022;162:635-46. [Crossref] [PubMed]
- Moons KG, Altman DG, Reitsma JB, et al. Transparent Reporting of a multivariable prediction model for Individual Prognosis or Diagnosis (TRIPOD): explanation and elaboration. Ann Intern Med 2015;162:W1-73. [Crossref] [PubMed]
- Ubuane PO, Animasahun BA, Njokanma OF. Dataset: PEF and FEV1 of Nigerian children aged 6-11 years measured with an electronic peak flow meter. Mendeley Data 2023; [Crossref]
- Ubuane PO, Ajiboye OA, Adekunle MO, et al. Reference values and equations for the 6-minute walk distance of Nigerian children aged 6-11 years: A cross-sectional study. Pediatr Pulmonol 2022;57:2103-15. [Crossref] [PubMed]
- Whittaker AL, Sutton AJ, Beardsmore CS. Are ethnic differences in lung function explained by chest size? Arch Dis Child Fetal Neonatal Ed 2005;90:F423-8. [Crossref] [PubMed]
- Goss-Sampson MA. Statistical Analysis in JASP: A Guide for Students v 0.16 (2022). 5th ed. 2022.
- Quanjer PH, Stanojevic S, Cole TJ, et al. Multi-ethnic reference values for spirometry for the 3-95-yr age range: the global lung function 2012 equations. Eur Respir J 2012;40:1324-43. [Crossref] [PubMed]
- Neder JA, Berton DC, O'Donnell DE. Calculating the statistical limits of normal and Z-scores for pulmonary function tests. J Bras Pneumol 2022;48:e20220182. [PubMed]
- Faleti AO. Lung function in children with and without sickle cell anaemia at the Lagos State University Teaching Hospital [dissertation]. Faculty of Paediatric, National Postgraduate Medical College of Nigeria. National Postgraduate Medical College of Nigeria, 2012.
- Bland JM, Altman DG. Statistical methods for assessing agreement between two methods of clinical measurement. Lancet 1986;1:307-10. [Crossref] [PubMed]
- Giavarina D. Understanding Bland Altman analysis. Biochem Med (Zagreb) 2015;25:141-51. [Crossref] [PubMed]
- Barton B, Peat J. Medical Statistics: A Guide to Data Analysis and Critical Appraisal. 2nd editio. West Sussex: John Wiley & Sons Ltd, 2014.
- Winkler C, Linden K, Mayr A, et al. RefCurv: A software for the construction of pediatric reference curves. Softw Impacts 2020;6:100040. [Crossref]
- Mittal S, Gupta S, Kumar A, et al. Regression equations for peak expiratory flow in healthy children aged 7 to 14 years from Punjab, India. Lung India 2013;30:183-6. [Crossref] [PubMed]
- Moffett AT, Bowerman C, Stanojevic S, et al. Global, Race-Neutral Reference Equations and Pulmonary Function Test Interpretation. JAMA Netw Open 2023;6:e2316174. [Crossref] [PubMed]
- Moaaz M, Badreldin O, El Chazli Y, et al. Race-neutral equations for assessment of lung function in children with thalassemia. Pediatr Pulmonol 2023;58:2543-50. [Crossref] [PubMed]
- Antalffy T, De Simoni A, Griffiths CJ. Promising peak flow diary compliance with an electronic peak flow meter and linked smartphone app. NPJ Prim Care Respir Med 2020;30:19. [Crossref] [PubMed]
- Côté J, Cartier A, Malo JL, et al. Compliance with peak expiratory flow monitoring in home management of asthma. Chest 1998;113:968-72. [Crossref] [PubMed]
- Faleti OA, Akode SO, Disu EA, et al. Pulmonary functions in children with sickle cell anaemia in steady state in Lagos, Nigeria. Ann Heal Res 2017;3:18-25.
- Mohammed J, Maiwada S, Sumaila F. Relationship between anthropometric variables and lung function parameters among primary school children. Ann Niger Med 2015;9:20. [Crossref]
- Mannino DM, Townsend MC. Spirometry in 2022: Is a Single Set of Prediction Equations for All the Best Path Forward? Am J Respir Crit Care Med 2023;207:659-61. [Crossref] [PubMed]
- Hüls A, Krämer U, Gappa M, et al. Age Dependency of GLI Reference Values Compared with Paediatric Lung Function Data in Two German Studies (GINIplus and LUNOKID). PLoS One 2016;11:e0159678. [Crossref] [PubMed]
- Mozun R, Ardura-Garcia C, Pedersen ESL, et al. Age and body mass index affect fit of spirometry Global Lung Function Initiative references in schoolchildren. ERJ Open Res 2022;8:00618-2021. [Crossref] [PubMed]
- Fawibe AE, Odeigah LO, Saka MJ. Reference equations for spirometric indices from a sample of the general adult population in Nigeria. BMC Pulm Med 2017;17:48. [Crossref] [PubMed]
- Madanhire T, Ferrand RA, Attia EF, et al. Validation of the global lung initiative 2012 multi-ethnic spirometric reference equations in healthy urban Zimbabwean 7-13 year-old school children: a cross-sectional observational study. BMC Pulm Med 2020;20:56. [Crossref] [PubMed]
- Pefura-Yone EW, Balkissou AD, Poka-Mayap V, et al. Spirometric reference equations for Cameroonians aged 4 to 89 years derived using lambda, mu, sigma (LMS) method. BMC Pulm Med 2021;21:344. [Crossref] [PubMed]
- Arigliani M, Canciani MC, Mottini G, et al. Evaluation of the Global Lung Initiative 2012 Reference Values for Spirometry in African Children. Am J Respir Crit Care Med 2017;195:229-36. [Crossref] [PubMed]
- Smith SJ, Gray DM, MacGinty RP, et al. Choosing the Better Global Lung Initiative 2012 Equation in South African Population Groups. Am J Respir Crit Care Med 2020;202:1724-7. [Crossref] [PubMed]
- Arigliani M, Lum S, Zuiani C, et al. Comparison of Lung Function in Healthy Nigerian Children Living in Nigeria and in the United Kingdom. Am J Respir Crit Care Med 2022;206:221-4. [Crossref] [PubMed]
- Sonnappa S, Lum S, Kirkby J, et al. Disparities in pulmonary function in healthy children across the Indian urban-rural continuum. Am J Respir Crit Care Med 2015;191:79-86. [Crossref] [PubMed]
- Akodu OS, Faleti OA, Adekanmbi AF, Adetutu T. Correlation of peak expiratory flow rate with anthropometric parameters in apparently healthy children with sickle cell anaemia in Lagos. Sri Lanka J Child Heal 2020;49:329–34.
- Sharma R, Kaur A, Gupta M, et al. A cross sectional study to assess peak expiratory flow rate in healthy school children for establishment of normative data. J Nepal Paediatr Soc 2020;40:1-6. [Crossref]
- Oloyede IP, Ekrikpo UE, Ekanem EE. Normative values and anthropometric determinants of lung function indices in rural Nigerian children: A pilot survey. Niger J Paediatr 2013;40:406-11. [Crossref]
- Akhiwu HO. Spirometric Values in Healthy Nigerian School Children Aged 6-11 Years. Journal of Advances in Medicine and Medical Research 2017;22:1-8. [Crossref]
- Gauld LM, Kappers J, Carlin JB, et al. Height prediction from ulna length. Dev Med Child Neurol 2004;46:475-80. [Crossref] [PubMed]
- Park JS, Suh DI, Choi YJ, et al. Pulmonary function of healthy Korean children from three independent birth cohorts: Validation of the Global Lung Function Initiative 2012 equation. Pediatr Pulmonol 2021;56:3310-20. [Crossref] [PubMed]
- Ferreira MS, Marson FAL, Wolf VLW, et al. Association between Pulmonary Function and Body Composition in Children and Adolescents with and without Obesity. J Clin Med 2022;11:7410. [Crossref] [PubMed]
- Bouti K, Benamor J, Bourkadi JE. Predictive Regression Equations of Flowmetric and Spirometric Peak Expiratory Flow in Healthy Moroccan Children. J Clin Diagn Res 2017;11:SC01-4. [Crossref] [PubMed]
- Martins C, Severo M, Silva D, et al. Development and validation of predictive equations for spirometry in Portuguese children. J Allergy Clin Immunol Glob 2023;2:100084. [Crossref] [PubMed]
- Okello G, Devereux G, Semple S. Women and girls in resource poor countries experience much greater exposure to household air pollutants than men: Results from Uganda and Ethiopia. Environ Int 2018;119:429-37. [Crossref] [PubMed]
- Martínez-Briseño D, Gochicoa-Rangel L, Torre-Bouscoulet L, et al. Comparing Spirometric Reference Values From Childhood to Old Age Estimated by LMS and Linear Regression Models. Arch Bronconeumol (Engl Ed) 2021;57:172-8. [Crossref] [PubMed]
- Chang SM, Tsai HJ, Tzeng JY, et al. Reference equations for spirometry in healthy Asian children aged 5 to 18 years in Taiwan. World Allergy Organ J 2019;12:100074. [Crossref] [PubMed]
Cite this article as: Ubuane PO, Ajiboye OA, Akinola AO, Adekunle MO, Akinyosoye G, Ajayi OA, Kayode-Awe MO, Ohagwu CI, Odusote OA, Animasahun BA, Njokanma FO. Development of reference values and equations for the pulmonary function of Nigerian children aged 6–11 years measured with digital peak flow meter and its validation against local and the Global Lung Function Initiative (GLI) equations. J Xiangya Med 2024;9:7.